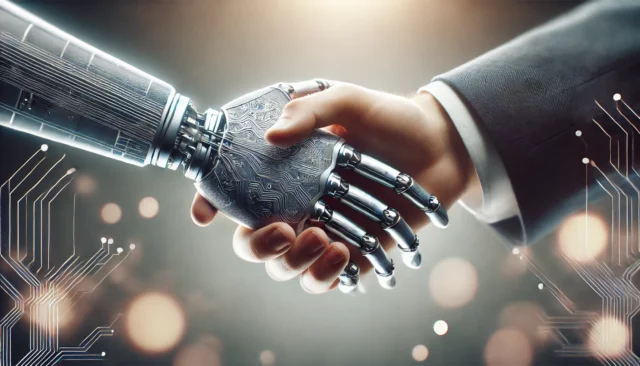
Despite the importance and timely arrival of the EU AI Act, major concerns remain about its compliance and impact on AI adoption and governance strategies. In fact, a recent survey found that having adequate AI governance in place is a top priority for 41% of business decision-makers. However, around a quarter of UK businesses have yet to do any AI preparation, partly due to continued confusion about their obligations.
The requirements under the law are specific, however, including for “companies or public authorities that develop or use AI applications that pose a high risk to the security or fundamental rights of citizens.” This high-risk category may include law enforcement and employment systems, as well as those used by life sciences and critical infrastructure organizations.
Fundamentally, organizations developing or adopting AI technologies must take a risk-based approach based on a range of considerations, from transparency and accountability to oversight and compliance.
In this context, one of the priorities that leaders must focus on is preparing their data, including which datasets they can and cannot use, their sensitivity and accuracy levels, among others. But this is just the beginning. Other crucial steps in the overall preparation process include creating policies that also meet compliance requirements. Remember, these systems must be trained to know what data is sensitive as well as the limits to apply when responding to a user request.
A three-point data preparation plan
The big question at this point, however, is how to proceed. What are the fundamental steps organizations should take to ensure their data is properly prepared for AI integration? Broadly speaking, there are three key priorities to address:
1 — Assess data maturity
The process should begin with a thorough assessment of existing data maturity levels. Key criteria include the extent to which the organization’s data sets are reliable and automated across all relevant areas, from financial and CRM data to inventory data. In particular, are these data sets consistent, reconciled, and reliable?
The assessment should also determine the extent to which manual, error-prone processes, such as those that rely on tools like Excel spreadsheets, are still being used. Organizations in this situation are less likely to be ready to adopt AI and will need to improve their data management before moving to the next step.
2 — Implement a modern data platform
Based on the results of the data maturity assessment, the next step is to create an action plan that prioritizes the most critical improvements needed to become AI-ready.
The focus here should be on building or improving a data platform that delivers reliable and relevant analytics. This platform should be able to fully automate data collection, processing, and reporting, while eliminating the need for manual intervention. This includes considerations such as moving from descriptive and diagnostic analytics (understanding what happened and why) to a situation where the organization can confidently base decisions on its data.
Organizations looking to update or replace their data platform should choose a solution that can scale with their needs, particularly one that can support more advanced analytics and AI as their data maturity level increases.
3 – Leverage AI and advanced analytics
Once the data platform is in place and providing reliable, automated insights, the organization is well positioned to begin leveraging AI and advanced analytics.
Assuming that the relevant datasets are reliable and well-managed, the process of developing advanced analytical models and AI-driven applications can begin. This can address a wide variety of requirements, from integrating AI into existing data systems to improve decision-making, to optimizing operations or providing predictive insights. In each situation, the data platform used for current analytics must also be able to support these advanced use cases.
The key takeaway is that organizations should systematically prepare their data infrastructure rather than jumping straight into AI integration without first doing the necessary preparatory work.
What is the emergency?
Among the various talking points that accompany the growth of AI is the need for urgency, which is also true for data strategy. For organizations that have the time and resources, this process should already be underway, and if it isn’t, it should begin immediately.
Of course, those who are slow to organize their data or who do not migrate to modern cloud-based platforms will certainly be able to come back to it in three to five years, for example. The problem is that at that point, they will be starting from scratch and will still have to go through the various important processes of data preparation and integration. They may also be competing with rivals who have acquired experience, momentum and a head start that could offer them a whole range of significant advantages.
The end point of true AI integration may therefore seem utopian, but as with everything, it can and must be broken down by organizations into steps to get there. They must address the fundamentals today to be able to take advantage of available AI technologies tomorrow.
Reuben Barry is Practice Director – Data & AI at Node 4.