In today’s fast-paced, data-driven world, it’s not uncommon to prioritize immediate growth over building slower-moving building blocks. But when it comes to adopting artificial intelligence (AI), the relentless pursuit of key performance indicators can set organizations up for short-term gains, but sometimes long-term losses. As we enter this new era of AI, the results of years of forgoing the creation of clean, consolidated data sets due to budget constraints and limited resources may begin to catch up with businesses.
Large Language Models (LLMs) are only successful if their data is clean. Centralized data observability platform Telmaï recently launched an experiment to better understand this. The results demonstrated that as the noise level in a dataset increases, the precision and accuracy gradually decrease, proving the impact of data quality on model performance. The experiment resulted in a drop from 89% to 72% in prediction quality with noise in the training data. LLMs require much smaller training data sets to achieve a certain quality when using high-quality data for fine-tuning. This results in reduced costs and development time.
The power of blank data
As more businesses integrate AI, the importance of data hygiene becomes even more pronounced. At the heart of AI applications, LLMs use large data sets to understand, summarize and generate new content, thereby increasing the value and impact of the data.
Organizations face potential risks when implementing AI applications, even if they forgo a stable database. Although these applications give more users access to data-driven insights – and more opportunities to act on that data – tackling data of fragile quality can lead to inaccurate results. It is best to provide users with robust analysis conducted on a solid, clean database.
Organizations can ensure the cleanliness of their data by establishing a single source of truth rather than multiple tables with similar data and slight discrepancies. If there is disagreement about the source of truth, there are likely valid claims that some aspects of each source are more reliable than others. This could be a matter of business rules applied as well as data quality management.
LLMs provide more flexibility in understanding and interpreting user questions, compared to needing to know exact field names or values in traditional queries and programming. However, these technologies find the best matches between imprecise user questions and available data and analytics. When you have a clear, clean database to map onto, technology is more likely to identify and present useful analysis. Uneven and unreliable data dilutes information and increases the likelihood of inaccurate or weak conclusions. When outliers appear in the data, they may be due to genuine performance changes or poor data quality. If you trust your data, you don’t need to spend as much time investigating potential data inaccuracies; you can jump straight into action with confidence when you’re sure the information accurately represents business realities.
Cleaner data, smarter decisions
When an organization collects data, it must define its purpose and apply data quality standards throughout its retention and analysis. Still, it’s worth cleaning or repairing your data to improve downstream analysis when data quality issues are identified.
Data cleaning is one of the most important steps to ensure that the data is ready for analysis. The process involves eliminating irrelevant data; this may include removing duplicate observations, correcting formatting errors, editing incorrect data, and handling missing data. Data cleansing is not just about erasing data, but finding ways to maximize its accuracy.
The first step to creating cleaner data is to determine the use case. Different organizations have different needs and goals. Some teams may want to predict trends, while others may focus on sustained growth and identify anomalies. Once the use case is determined, data teams can begin to evaluate the type of data needed to perform the analysis and correct structural errors and duplicates to create a cohesive data set.
Data priority matrices can help prioritize which errors to address first and the level of difficulty. Each data problem can be rated on a scale of one to five, with one being the least serious and five being the most serious. Fixing easy-to-change errors first can make a noticeable difference without spending a lot of time or resources. It’s also helpful to define “good enough” and not spend too many resources chasing perfection with diminishing returns. Sometimes a model can be about as robust with a data completeness rate of 98% versus a data completeness rate of 99.99%. It is good to evaluate the effort holistically between data engineers, data scientists and business users to determine whether it is better to spend the efforts on the last part of the data rather than moving to another dataset or other functionality.
It is important to keep in mind the consequences of acting on incorrect or incomplete data in each area. Certain attributes may be a key detail for the use case, such as the channel through which a customer engages. Other attributes may be valid, but relatively insignificant, indicators, such as the version of a web browser through which a customer engages.
Conclusion
Data cleanliness is an often overlooked best practice that has been tolerated by businesses for decades. However, with the The AI market is expected to grow twentyfold by 2030, the need for clean data has come into the spotlight given the interdependence with AI results. Data teams should take advantage of this opportunity and C-suite attention to advocate for establishing a standardized data collection process to prioritize clean data as early as possible. This priority will enable organizations to better protect their data assets and unlock the full potential of AI.
About the Author
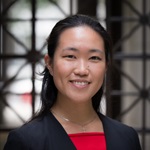
Stephanie Wong is Director of Data and Technology Consulting at DataGPT, the leading provider of conversational AI data analysis software. Formerly with Capgemini and Slalom Consulting, Stephanie is a seasoned data consultant with an unwavering commitment to creativity and innovation. She has helped Fortune 500 companies derive more value and insights from their data and has over a decade of experience spanning the entire data lifecycle, from data warehousing to learning automatic and executive dashboards.
Sign up for free at insideBIGDATA newsletter.
Join us on Twitter: https://twitter.com/InsideBigData1
Join us on LinkedIn: https://www.linkedin.com/company/insidebigdata/
Join us on Facebook: https://www.facebook.com/insideBIGDATANOW