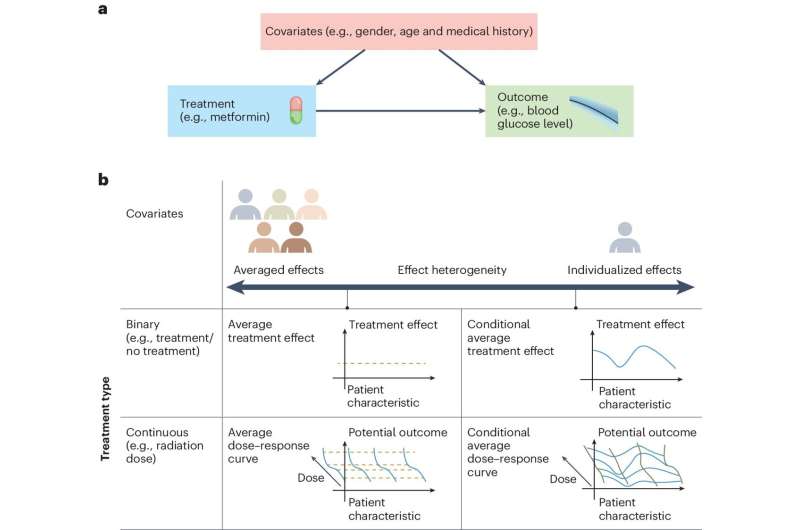
Formalization of tasks for causal ML. Credit: Natural medicine (2024). DOI: 10.1038/s41591-024-02902-1
Artificial intelligence is making progress in the medical field. In terms of imaging techniques and calculation of health risks, there is a plethora of AI methods in the development and testing phase. Wherever it comes to recognizing patterns in large volumes of data, machines are expected to bring great benefits to humanity. Following the classic model, AI compares information to learned examples, draws conclusions and makes extrapolations.
Now, an international team led by Professor Stefan Feuerriegel, director of the Institute of Artificial Intelligence (AI) in Management at LMU, is exploring the potential of a relatively new branch of AI for diagnosis and therapy . Can causal machine learning (ML) estimate treatment outcomes – and do it better than machine learning methods typically used so far? Yes, says a study by the group, which was published In Natural medicine and is entitled “Causal ML can improve the effectiveness and safety of treatments”.
In particular, the new ML variant offers “many opportunities to personalize treatment strategies and thus individually improve the health of patients,” write the researchers from Munich, Cambridge (United Kingdom) and Boston (United States). Stefan Bauer and Niki Kilbertus, professors of computer science at the Technical University of Munich (TUM) and group leaders at Helmholtz AI.
Regarding automatic assistance with therapeutic decisions, the authors anticipate a decisive leap forward in terms of quality. Classic ML recognizes patterns and discovers correlations, they argue. However, the causal principle of cause and effect generally remains closed to machines; they cannot answer the question why. And yet, many questions that arise when making treatment decisions contain causal issues within them.
The authors illustrate this with the example of diabetes: classical ML would aim to predict the probability of a disease for a given patient with a range of risk factors. With causal ML, it would ideally be possible to determine how the risk changes if the patient receives an antidiabetic drug; that is to say, evaluate the effect of a cause (prescription of a medication). It would also be possible to estimate whether another treatment plan would be better than, for example, the commonly prescribed drug metformin.
However, to be able to estimate the effect of a hypothetical treatment, “AI models must learn to answer questions like “What if?” nature”, explains Jonas Schweisthal, doctoral student in Feuerriegel’s team.
“We give the machine rules to recognize the causal structure and correctly formalize the problem,” Feuerriegel explains. Then the machine must learn to recognize the effects of interventions and understand, so to speak, how the real consequences are reflected in the data fed into the computers.
“The software we need for causal ML methods in medicine does not exist directly,” says Feuerriegel. Rather, “complex modeling” of the problem in question is required, involving “close collaboration between AI experts and doctors”.
Like his TUM colleagues Stefan Bauer and Niki Kilbertus, Feuerriegel also studies questions related to AI in medicine, decision-making and other topics at the Munich Center for Machine Learning (MCML) and the Konrad Zuse School of Excellence in Trusted AI.
In other application areas, such as marketing, Feuerriegel explains, work with causal ML has already been in the testing phase for several years. “Our goal is to bring methods closer to practice. The document outlines the direction in which things could develop in the coming years.”
More information:
Feuerriegel, S., et al. Causal machine learning to predict treatment outcomes. Natural medicine (2024). DOI: 10.1038/s41591-024-02902-1
Provided by
Ludwig Maximilian University Munich
Quote: Researchers explore causal machine learning, a new advance for AI in healthcare (April 19, 2024) retrieved October 4, 2024 from https://medicalxpress.com/news/2024-04-explore-causal -machine-advancement-ai. HTML
This document is subject to copyright. Except for fair use for private study or research purposes, no part may be reproduced without written permission. The content is provided for informational purposes only.