The 2024 Nobel Prize in Chemistry honors the transformative impact of AI on protein science, with advances expected to reshape drug development.
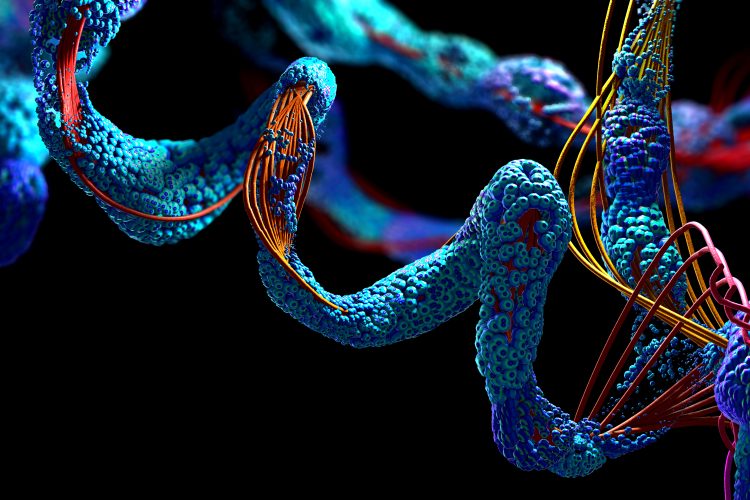
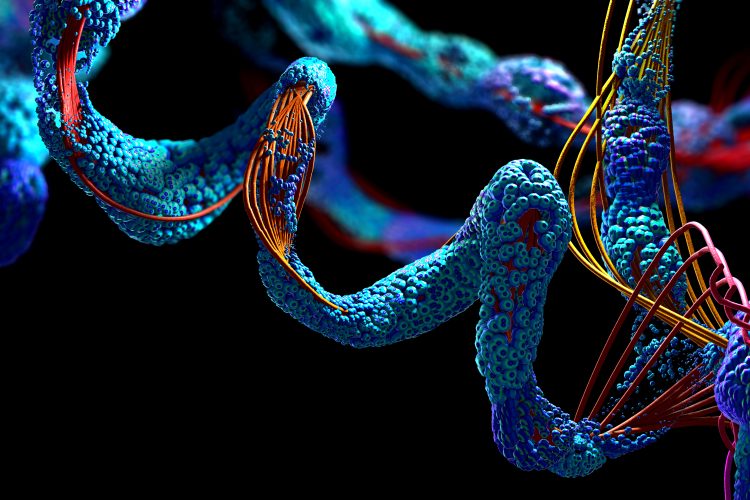
The 2024 Nobel Prize in Chemistry has been awarded to Demis Hassabis and John Jumper of Google DeepMind for the development of AlphaFold2, and to David Baker of University of Washington for his work on computational protein design. These innovations have revolutionized the understanding of protein structures thanks to artificial intelligence.
Before AlphaFold, determining 3D protein structures was complex and time-consuming, and often required the use of experimental methods such as X-ray crystallography and cryo-electron microscopy. AlphaFold is a neural network-based model, powered by a deep learning algorithm that integrates large amounts of physical and biological data on protein structures. By leveraging multiple sequence alignments, it can predict protein structures with near-experimental accuracy. Since its launch, AlphaFold has accurately predicted the structure of more than 200 million proteins and has been used by more than 2 million researchers worldwide. Despite its recent development, AlphaFold2 has already been applied in numerous studies in biology and medicine. Its applications include studying disease pathology to develop targeted therapies, visualizing enzymes capable of breaking down plastics, and engineering solutions to antibiotic resistance, among others.
The second half of the prize went to David Baker, a structural biologist at the University of Washington, for his research on computational protein design. Baker and his team developed Rosetta, an algorithm for ab initio protein structure prediction, that is, designing entirely new proteins from scratch. The Rosetta algorithm has been integrated into AI-based tools like RoseTTAfold, which uses a three-track neural network to predict protein structures. Baker’s model could revolutionize drug development, synthetic biology and nanotechnology, paving the way for new medical treatments, vaccines, nanomaterials and sensors. Key applications include designing proteins to mimic those involved in photosynthesis for renewable energy, creating molecular detectors that activate when detecting cancer cells, and engineering proteins into microscopic “nanocages” for carry medications through the body.
AI-based inventions
This is the first time a Nobel Prize has been awarded for an AI-based scientific breakthrough, and it likely won’t be the last.
AI is leveraged across scientific fields to identify meaningful trends in large data sets, predict outcomes based on that data, and simulate complex physical and biological scenarios. AI is influencing all sectors, but its impact will be particularly strong in the pharmaceutical sector where it is expected to accelerate drug discovery and, in clinical settings, generate models and insights from large amounts of patient data to enable more personalized treatments. As the life sciences and pharmaceutical industries increasingly rely on AI-driven discoveries, companies operating in this space will need to think carefully about how to achieve meaningful protection for both AI platforms and for the products generated by these platforms.
AI inventions as intellectual property
Although DeepMind has made AlphaFold2’s code open source, allowing for widespread use and collaboration, its underlying methods are protected by patents. DeepMind filed three international (PCT) applications in 2019, which cover relatively broad methods of generating multiple structural predictions, updating parameters using neural networks, and selecting the final structure.
At the European Patent Office (EPO), an invention must represent a non-obvious technical solution to a technical problem to be patentable. With this in mind, it may seem tricky to patent the “core of AI”, that is to say the fundamental elements of an AI model, to the extent that these algorithms are often considered by the EPO as abstract mathematical methods devoid of technical effect. However, with the right guidance, certain aspects of AI inventions can be patented. These aspects include how the training data is generated for use in training an AI model, how the AI model is trained using the training data, and how whose model analyzes the data. A clear link must be established between these aspects and a technical effect or advantage – and the technical objective must be plausibly achieved within the full scope of the patent’s claims.
Compounds discovered through AI are a more obvious target for patent protection. David Baker is listed as an inventor on numerous patents, focusing on compositions and methods of treating particular diseases. Presumably, some of these compositions include proteins synthesized using Baker’s AI model. Another example is Benevolent AIa company collaborating with AstraZeneca to improve both drug development and reuse through AI – they have several patents for their AI model’s products (compounds and uses thereof).
Whether it is a product of an AI or the AI itself, obtaining a patent is advantageous because it gives the owner exclusive rights to make, use, sell or to license his invention for a specified period. Through these exclusive rights, they can establish a competitive advantage, control market entry, and potentially set higher prices due to lack of competition. Patents can attract investors by ensuring intellectual property protection. This strengthens the reputation of the inventor, making it easier to obtain funding for development and commercialization. Finally, because patents require public disclosure of the invention, the inventor can inspire future innovation while retaining his or her exclusive rights.
Patent or trade secret?
Alternatively, certain aspects of an AI-based invention may be retained as trade secrets. Unlike patents, which require public disclosure of the invention, trade secrets remain private. This can be advantageous in certain scenarios, for example where the invention is not eligible for patent protection, or in extremely rapidly changing industry sectors where the risk of obsolescence outweighs the benefits of patenting.
However, protecting trade secrets relies heavily on the ability to maintain confidentiality by enforcing internal security measures or non-disclosure agreements (NDAs). If the trade secret is leaked or leaked, its protection is lost, which can cause significant damage to the company. Additionally, trade secrets provide no protection against independent discovery or reverse engineering by competitors. This means that trade secrets are often more useful as a complement to other forms of intellectual property protection, for example patents, rather than as a stand-alone solution.
In the case of AlphaFold2, although much of the system is public, some details about algorithms, neural network architecture or data processing processes could be kept as trade secrets. In many areas of technology, companies often use a combination of patents and trade secrets to maximize protection for their innovations.
About the author
Roberta Sher, technical assistant at Reddie & Grose
Roberta Sher is a technical assistant in the Life Sciences team at intellectual property law firm Reddie & Grose. She joined the firm in September 2022 after completing an integrated master’s degree in chemistry at the University of Oxford. Roberta also has experience working in clinical and regulatory affairs for a medical device startup that developed a non-invasive device for objective measurement of pain during surgery.