New expert panel analyzes emerging architectures for AI models
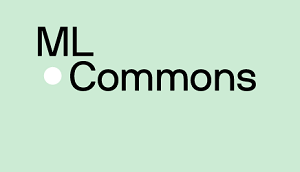
Today, MLCommons® announced new results for its industry-leading MLPerf testing tool®Inference Benchmark Suite v4.1, which provides performance testing of machine learning (ML) systems in an architecture-neutral, representative, and reproducible manner. This release includes the first results of a new benchmark based on a mixed model of experts (MoE) architecture. It also presents new findings on the energy consumption of inference execution.
MLPerf Inference v4.1
The MLPerf inference benchmark suite, which spans both data centers and edge systems, is designed to measure how quickly hardware systems can run AI and ML models in a variety of deployment scenarios. This open-source, peer-reviewed inference benchmark suite creates a level playing field that drives innovation, performance, and energy efficiency across the industry. It also provides critical technical insights for customers purchasing and optimizing AI systems.
Benchmark results from this round demonstrate broad industry participation and include the launch of six newly available or soon-to-ship processors:
○ AMD MI300x Accelerator (Available)
○ AMD EPYC “Turin” Processor (Preview)
○ Google “Trillium” TPUv6e Accelerator (Preview)
○ Intel “Granite Rapids” Xeon Processors (Preview)
○ NVIDIA “Blackwell” B200 Accelerator (Preview)
○ UntetherAI Accelerators SpeedAI 240 Slim (available) and SpeedAI 240 (preview)
MLPerf Inference v4.1 includes 964 performance results from 22 participating organizations: AMD, ASUSTek, Cisco Systems, Connect Tech Inc, CTuning Foundation, Dell Technologies, Fujitsu, Giga Computing, Google Cloud, Hewlett Packard Enterprise, Intel, Juniper Networks, KRAI, Lenovo, Neutral Magic, NVIDIA, Oracle, Quanta Cloud Technology, Red Hat, Supermicro, Sustainable Metal Cloud and Untether AI.
“There are now more choices than ever when it comes to AI systems technologies, and it’s encouraging to see vendors embrace the need for open and transparent performance criteria to help stakeholders evaluate their technologies,” said Mitchelle Rasquinha, co-chair of the MLCommons Inference Working Group.
New Expert Reference Blend
Adapting to today’s evolving AI landscape, MLPerf Inference v4.1 introduces a new benchmark to the suite: MoE. MoE is an architectural design for AI models that departs from the traditional approach of using a single massive model; instead, it uses a set of smaller “expert” models. Inference queries are directed to a subset of the expert models to generate results. Research and industry leaders have found that this approach can produce accuracy equivalent to a single monolithic model, but often with a significant performance advantage because only a fraction of the parameters are invoked with each query.
The MoE benchmark is unique and one of the most complex implemented by MLCommons to date. It uses the open source Mixtral 8x7B model as a reference implementation and performs inference using datasets covering three independent tasks: general question answering, mathematical problem solving, and code generation.
“When deciding to add a new benchmark, the MLPerf Inference Working Group observed that many key players in the AI ecosystem were heavily adopting MoE as part of their strategy,” said Miro Hodak, co-chair of the MLCommons Inference Working Group. “Creating an industry-standard benchmark to measure system performance on MoE models is critical to addressing this trend in AI adoption. We are proud to be the first AI benchmark suite to include MoE testing to fill this critical information gap.”
Comparative analysis of energy consumption
The MLPerf Inference v4.1 benchmark includes 31 power consumption test results across three submitted systems spanning both data center and edge scenarios. These results demonstrate the continued importance of understanding the power requirements of AI systems running inference tasks, as power costs represent a substantial portion of the overall operating expenses of AI systems.
The Increasing Pace of AI Innovation
Today, we are seeing an incredible wave of technological advancements across the AI ecosystem, driven by a wide range of vendors, including AI pioneers, large, established technology companies, and small startups.
MLCommons particularly welcomes new MLPerf Inference submitters AMD and Sustainable Metal Cloud, as well as Untether AI, who have delivered results in both performance and energy efficiency.
“It’s encouraging to see the breadth of technical diversity in the systems tested in the MLPerf Inference benchmark, as vendors adopt new techniques to optimize system performance, such as vLLM and sparsity-aware inference,” said David Kanter, lead of MLPerf at MLCommons.
“Further down the technology stack, we were struck by the substantial increase in unique acceleration technologies submitted for benchmarking this time around. We are excited to see that systems are now evolving at a much faster pace – at every level – to meet the needs of AI. We are excited to be a trusted provider of open, fair and transparent benchmarks that help stakeholders get the data they need to understand the rapid pace of AI innovation and move the industry forward.”
See the results
To view MLPerf Inference v4.1 results, please visit HERE.
Subscribe to insideAI news for free newsletter.
Join us on Twitter: https://twitter.com/InsideBigData1
Join us on LinkedIn: https://www.linkedin.com/company/insideainews/
Join us on Facebook: https://www.facebook.com/insideAINEWSNOW