It should come as no surprise to anyone that financial services institutions have a treasure trove of data. Generated by consumer interactions, market movements and the ebb and flow of internal operations, these statistics – if harnessed correctly – can have a transformative impact on the fortunes of the company. With the right distillation of data, a CFO could find new ways to close a quarter early, a logistics chief could straighten out an increasingly complex supply chain, or a CIO would finally understand the best way to build products personalized financial services for its bank’s growing army of customers.
Phrases like that, of course, are the reason for the enduring popularity of the phrase “it’s easier said than done.” Harness the power of data for a bank or fintech, that means finding a way to turn millions or even billions of data points into models and forecasts that are truly useful for your average industry CEO or CIO. It requires more than a deft hand with Excel spreadsheets: it requires a thoughtful, holistic strategy to automate the process of discovering and analyzing your company’s data at scale.
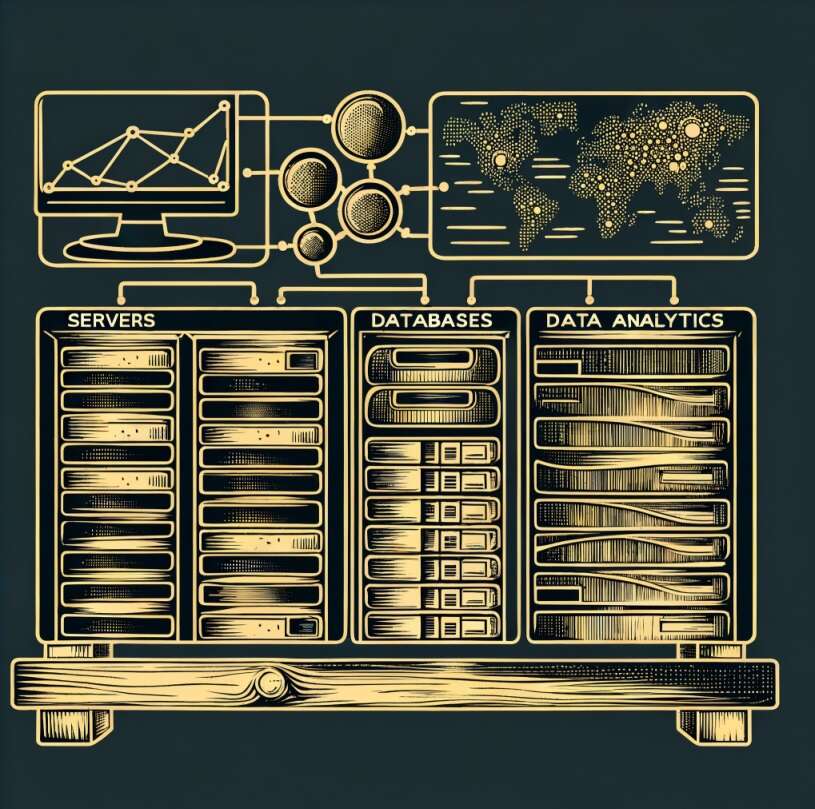
The Right Data Analytics Stack
The technologies available to CIOs to modernize the data management journey across the stack have never been better. Evidence of this is the growing importance of cloud-based data warehouses, as well as the confidence shown by financial services IT teams over the past year to quickly create their own LLMs based on internal training data.
Despite this clear trajectory toward data maturity, many organizations are simply unable to unlock the full potential of their data to ensure it drives business value, even as its volume and variety continues to grow. According to the European Commission, 80 percent of data collected in industry remains unused. Many still overspend on the cloud, or find themselves saddled with a proliferation of tools and purchasing unused software licenses. Simply put, many organizations’ data analytics stacks are not set up to take advantage of available technologies.
A data analytics stack is necessary to more effectively address the different roles and use cases found in today’s financial services industry. It should have the flexibility to support multiple deployment scenarios: for example, managing data pipelines, whether on-premises, private cloud, public cloud, multi-cloud, or hybrid. Likewise, it should allow operators to transform their data into any data warehouse environment of their choice and allow them to create a data workflow in one place and run it in another.
Perhaps most importantly, a good data analytics stack should enable a company’s staff to get the most out of its technologies. It should be accessible – ideally low-code or even no-code – to allow anyone, not just data scientists or engineers, to easily compile and analyze data. Whether it’s developing personalized offers for customers, reducing churn, or ensuring regulatory compliance, every line of business must have the ability to creatively solve its own analytical problems and apply own domain knowledge to relevant and impactful use cases.
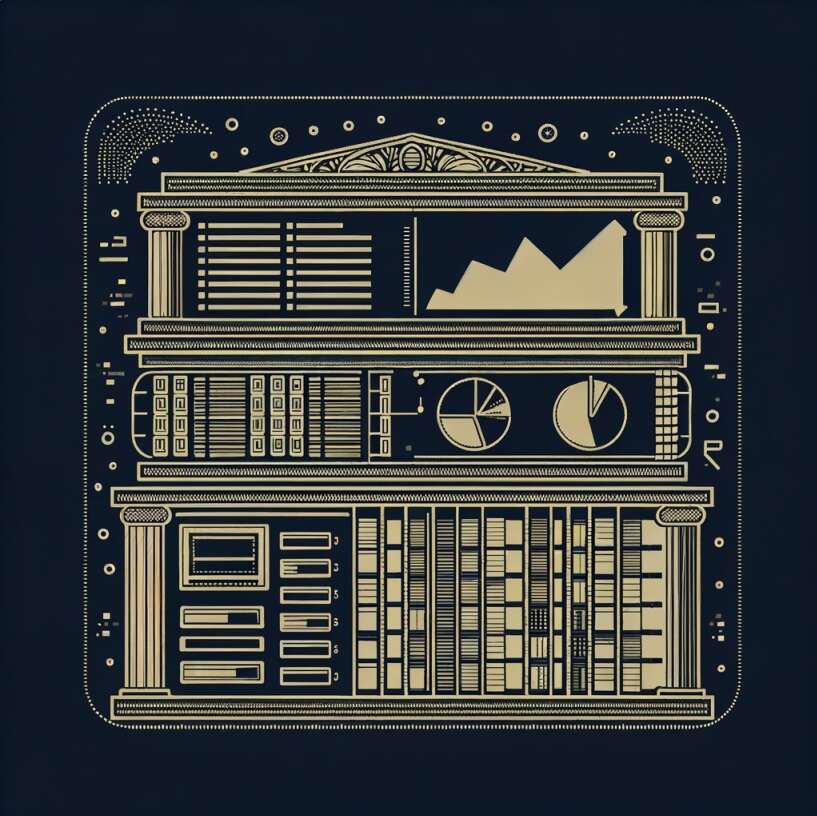
Automation to support regulatory compliance
To illustrate just one of the benefits of a technology stack that can automate data analysis, consider how it can be used to ensure regulatory compliance.
Regulations such as MiFIR and MiFID II require financial institutions to report applicable transactions. But given that millions of transactions can take place every minute – especially at large institutions – manually preparing and cleaning data for quality assurance (QA) purposes can be an extremely slow process. As a result, it can take up to two months before relevant regulators are notified, exposing financial institutions to regulatory fines if any part of the system fails that requires correction.
An automated data analytics stack, combining data engineering capabilities with a mix of visual analytics tools, can be used to create a streamlined workflow that alerts institutions in real time if they need to make corrections to ensure compliance. This transforms the quality assurance process from reactive to proactive, allowing teams to resolve issues as they arise rather than waiting months for results and thereby mitigating the risk of potentially costly errors .
This is just an example. Automated data analytics can be applied to almost any business use case, enabling financial services institutions to deploy an analytics strategy to identify new opportunities, more easily comply with regulations, meet ESG criteria, And much more.
In this new era of data value, a modern, automated data analytics stack with a simple, accessible interface will enable everyone within a financial services company to deliver this value to stakeholders. inside and outside their organization.