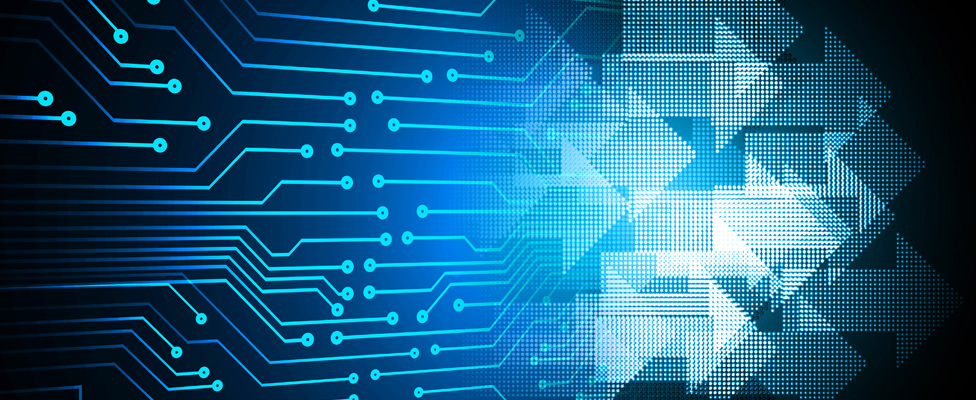
Is it too late for my organization to take advantage of AI?
The good news is that it’s not too late to start leveraging AI, but time is running out.
Business leaders have spent years being inundated with information about emerging applications of AI. As innovations like generative AI and large language models (LLM) become more popular, thoughts about the death of manual labor are becoming more common. In this rapidly changing environment, many business leaders are asking themselves the following questions: Is it too late for my organization to competitively leverage AI?
The short answer is no, but a pragmatic approach to AI adoption is becoming increasingly valuable.
Enthusiasm for AI is still in its infancy
The 2023 Gartner Hype Cycle placed generative AI at the “peak of inflated expectations”“, beyond the technology’s initial trigger point but before the trough of disillusionment (during which enthusiasm for AI may temporarily fade). This is important because early applications of AI AI are not always realistic and enterprise AI is not yet ubiquitous. According to Altair, most organizations have not even implemented AI at scale. More than a third (36%) ) business leaders we hope to adopt AI in the next six months; Another 23% plan to do so in the next year.
The AI track remains open. However, leaders looking to leverage AI should prioritize responsible AI use and robust data management as soon as possible.
Laying the foundations for responsible AI
Unregulated AI exposes organizations to several risks, including:
- Ethical concerns, such as bias and discrimination
- Security risks, including potential data breaches and insecure cyber protections
- Accountability issues, especially if AI stakeholders are not aligned on the technology’s use cases or desired impact
According to McKinsey, only 32% of organizations have attempted to mitigate AI inaccuracies, and only 28% are addressing cybersecurity risks. This surveillance is more than a missed opportunity: it threatens business continuity. Such statistics may explain why almost half (42%) of Altair survey respondents experienced failures. AI implementations over the past two years.
These potential downsides should not deter leaders from fully implementing AI. After all, the risks of not AI implementation is also severe, with high-performance AI implementations capable of improve the productivity of an individual worker by 40%. This boost gives organizations a competitive advantage, especially in a business environment plagued by labor shortages.
The key to effective AI implementation is caution and planning. Leaders should assess their company’s organizational, operational, and business challenges and use these findings to guide an intelligent AI strategy.
- Organizationally, successful AI implementation requires interdepartmental collaboration and training. Stakeholders – including leaders and daily drivers of productivity – need to understand the benefits of implementing AI. Otherwise, employee anxieties or misinformation could hinder progress.
- Operational challenges in deploying AI include inefficient manual processes and a lack of standardization. Remember, AI is not a silver bullet for solving existing technological inefficiencies. Before implementation, leaders should assess their technology stack, ensuring that all relevant software is in conversation with each other.
- From a business perspective, unclear use cases for AI are a recipe for disaster. Investments in AI and machine learning (ML) should have specific KPIs. Additionally, all investments should take a phased approach that prioritizes a solid database before deployment.
Managing data to enable AI success
High-quality data engenders organizational trust and enables better decision-making. According to an IDC study, reliable data facilitates better customer satisfaction and better innovationleading to benefits such as a potential 29% increase in revenue and a 31% reduction in operational costs.
Good data management practices, including regular audits, master data management (MDM) solutions, and workplace training, can significantly improve AI outcomes. When equipped with accurate, timely, and complete data, AI systems draw better conclusions and suggest more beneficial organizational improvements. Conversely, AI systems that rely on inaccurate data can make misleading suggestions when making critical business decisions.
A high-quality data repository should be centralized and not duplicative. In other words, all stakeholders should be able to quickly access relevant information and data should not have unnecessary copies (excluding security-related backups). In addition to the expense of storing duplicate data, multiple (and scattered) versions can disrupt and overburden AI systems, not to mention human workers.
The future is artificial
Humans will remain the primary drivers of organizational results, but there is no doubt that the future of business lies in AI-enabled collaborative work. As more leaders turn to AI to solve costly problems like inefficiency and labor shortages, the message for holdouts is clear: There is no better better time than today to start developing an AI plan. Fortunately, with the right technology stack, attitude, and data management strategy, leaders who start now position themselves to achieve tangible, remarkable results over the next two years.
About the Author
Brett Hansen is the CGO of Semarchia where he is responsible for go-to-market operations, including marketing, business development, and alliances and partnerships. Before joining Semarchy, he was CMO at Logi Analytics, a company acquired by Insight Software. He spent 11 years at Dell as an executive, responsible for software products and GTM within the Dell Client Group, and before that he worked at IBM in various marketing and channel leadership roles. You can reach the author via E-mail or via LinkedIn.