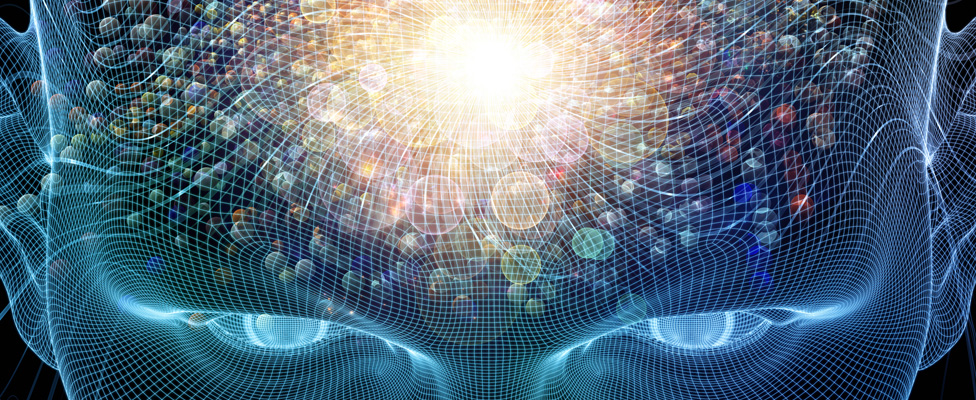
Insights into data and the role of AI with Philip Zelitchenko
Organizations can overcome barriers to obtaining timely information. ZoomInfo’s Philip Zelitchenko explains how AI can help.
As part of TDWI’s three-part podcast series examining TDWI’s latest Best Practices Report (BPR) on reducing time to insight and maximizing the benefits of real-time data, Philip Zelitchenko, vice president of data and analytics at ZoomInfo, offers his point of view on barriers to accessing information, strategies for improving information (and the role of data quality), and the use of AI (and its future). (Editor’s note: Quotes from speakers have been edited for length and clarity.)
The conversation began with a discussion of the obstacles faced by organizations trying to get better information faster. Zelitchenko identified several obstacles, starting with silos.
“When your data exists in multiple places within the organization, you have data that resides on the business side. You have systems like your CRM application and operational systems that reside on the product side. When a visitor lands on your website, all these engagements are recorded in different areas, both on the business side and on the product side.
Zelitchenko suggests that your business should first determine how quickly it will need this information. The answer, he says, partly depends on whether you’re a B2B or B2C company. “I point this out because data freshness and real-time or near-real-time applications are different for different use cases in these two types of businesses. Once the data from these different systems is brought together in one centralized location, the question then becomes: how often do I need this updated data and for what purpose? In the B2C world, time to information is critical. People come to your website, go to Amazon and look at different products. You’re trying to capture the buyer right now. In the B2B world, this varies. Sellers in a B2B world can leverage the information and signals captured in a higher latency environment.
What is the best strategy for addressing data quality and trust issues to obtain the best possible insights? Zelitchenko gave an example to illustrate some of the different challenges caused by poor data quality: Many B2B companies don’t understand who their customer is.
“Suppose our company has 16 Google accounts in our CRM system. There are 16 different sub-teams at Google that use our product. We need to be able to capture all of these instances and group them into a single group. The ability to see that, the ability to understand that within our total addressable market, under a company called Google, there are multiple buying groups is important. Being able to distinguish which ones are relevant, which ones match our ideal customer profiles, this ability is essential to our ability to enter the market very effectively. This is a big piece of the puzzle that needs to be solved.
How do you get there? “At ZoomInfo, the tools we use vary by group. In some cases, real-time usage and data quality are important, which is why we implement our own solution. There are other cases where the data is not real-time, and we use other tools to address those use cases, monitor that data, and make sure it doesn’t change often. Different products, different use cases, but we try to monitor data quality throughout the funnel.
Among the current tools of interest in various fields, including data analysis and insights, is artificial intelligence. What is the impact of AI, including generative AI? Can this help generate insights faster?
The latest innovations concern LLMs, according to Zelitchenko, who gives as an example its Copilot product that the company will launch soon. “LLMs and generative AI will impact the efficiency and productivity of each individual contributor. If you look at our Copilot, for example, it gives account managers and others the ability to provide higher quality coverage because parts of their work are automated. AI provides greater coverage on the accounts they cover and provides better quality of coverage.
“Another example is another copilot product: Microsoft Copilot. His co-pilot helps software engineers be more efficient in the code they write. We saw about a 26-27% improvement. Our CTO just talked about it on social media compared to other companies talking about a 40% improvement. Does this mean we need fewer people to write code? No, because what’s happening is that the tide lifts all boats – and now every business benefits from this efficiency gain.
Improving the efficiency of all employees means that “we are now able to generate more code, which means releasing more features and creating more products with higher quality in the long term, because we can accelerate a part of the work we do. That’s great!”
Implementing co-pilots is not without its own challenges. The LLM co-pilots used are based on the data you provide them, but the quality of that data is essential. “Most LLMs suffer from hallucinations – the amount varies from very good patterns (around 4-5% hallucinations) to poorer patterns (around 12-14%). In these cases, you need better data governance to ensure that the quality of the data coming out is valid and can be used for production. It may be years before we get those LLMs where these hallucinations are reduced to 1%. It seems like 4 or 5% is a pretty good number, but if you’re operating on a larger scale it can add up pretty quickly.
In the future, does Zelitchenko expect each company to have its own large personal language model operating within its own organization? “The industry is evolving quite quickly. Improvements are happening on a large scale every week, and the cost of servicing and training these LLMs is dropping significantly. In a year or two, the ability of a business of any size to operate according to its own models will become a given and a commodity. You can also see this based on the open source approach many companies are taking, which will allow anyone to take a model and train it.
To get started with this technology, Zelitchenko recommends the agile method. “Start with something small. Try it and make sure it works. Test it on your clients in small groups. Make sure employees play with it.
(Editor’s note: You can listen to the podcast on demand here.)