Companies exploring the implementation of AI, which constitutes the major part companies from 2024— are currently evaluating ways to do this safely and sustainably. AI ethics can be a critical part of this discussion. The following questions are of particular interest:
- How diverse or representative is the training data for your AI engines? How can a lack of representation impact AI results?
- When should AI handle a sensitive task rather than a human? What level of oversight should organizations put in place over AI?
- When and how should organizations inform stakeholders that AI has been used to accomplish a certain task?
Organizations, especially those operating proprietary AI engines, need to answer these questions thoroughly and transparently to satisfy all stakeholder concerns. To help with this process, let’s review some pressing developments in AI ethics over the past six months.
The Rise of Agentic AI
We are quietly entering a new era of AI. “Agentic AI,” as it is called, can act as an “agent” that analyzes situations, uses other technologies to make decisions, and ultimately arrives at complex, multi-step decisions without constant human oversight. This level of sophistication sets agentic AI apart from the versions of generative AI that have emerged on the market that couldn’t tell users the time or add up simple numbers.
Agentic AI systems can process and “reason” about a complex dilemma with multiple criteria. For example, you are planning a trip to Mumbai. You want the trip to coincide with your mother’s birthday, and you want to book a flight that will allow you to benefit from your reward miles. Additionally, you want a hotel close to your mother’s house, and you want to make reservations for a nice dinner on the first and last night of your trip. Agentic AI systems can integrate these disparate needs and come up with a feasible itinerary for your trip, then book your stay and travel, interfacing with multiple online platforms to do so.
These capabilities will likely have huge implications for many businesses, especially data-intensive industries like financial services. Imagine being able to synthesize, analyze, and query your AI systems on your customers’ activities and profiles in just a few minutes. The possibilities are exciting.
However, agentic AI also raises a crucial question about AI oversight. Booking travel may be harmless, but other tasks in compliance-driven industries may require setting parameters on how and when AI can make executive decisions.
New compliance frameworks
Financial institutions now have an opportunity to codify some expectations around AI, with the goal of improving customer relationships and proactively prioritizing their customers’ well-being. Areas of focus in this regard include:
- Safety and security
- Responsible development
- Prejudice and illegal discrimination
- Confidentiality
While we cannot guess the timing or likelihood of regulation, organizations can conduct due diligence to help mitigate risks and underscore their commitment to customer outcomes. Important considerations include AI transparency and consumer data privacy.
Risk-based approaches to AI governance
Most AI experts agree that a one-size-fits-all approach to governance is insufficient. After all, the ramifications of unethical AI differ greatly depending on the application. That’s why risk-based approaches, such as those adopted by the EU’s comprehensive AI law— are gaining ground.
In a risk-based compliance system, the strength of punitive measures depends on the potential impact of an AI system on human rights, safety, and societal well-being. For example, high-risk sectors such as healthcare and financial services could face greater scrutiny regarding the use of AI, as unethical practices in these sectors can have a significant impact on consumer well-being.
Companies in high-risk industries must remain particularly vigilant about the ethical deployment of AI. The most effective way to do this is to prioritize human-involved decision-making. In other words, humans must have the final say when validating results, checking for bias, and enforcing ethical standards.
How to reconcile innovation and ethics
Discussions about AI ethics usually refer to the need for innovation. These phenomena (innovation and ethics) are described as opposing forces. However, I believe that incremental innovation need a commitment to ethical decision-making. When we rely on ethical systems, we create more sustainable, long-term, and inclusive technologies.
Perhaps the most important consideration in this area is explainable AI, or systems with decision-making processes that humans can understand, audit, and explain.
Many AI systems currently operate as “black boxes.” In short, we can’t understand the logic behind the outputs of these systems. Unexplainable AI can be problematic when it limits the ability of humans to verify—intellectually and ethically—the accuracy of a system’s logic. In these cases, humans can’t prove the truth of an AI’s response or action. Perhaps even more troubling, unexplainable AI is harder to iterate on. Leaders should consider prioritizing the deployment of AI that humans can regularly test, verify, and understand.
The balance between ethics and innovation in AI may seem delicate, but it is nonetheless essential. Leaders who question the ethics of their AI vendors and systems can improve their longevity and performance.
About the author
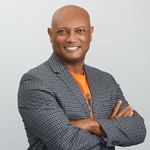
Vall Herard is the CEO of ItsIen.aia Fidelity Labs company. He brings a wealth of experience and expertise in the field and can shed light on where the industry is headed and what industry players should expect for the future of AI. Throughout his career, he has seen the evolution of AI use in the financial services industry. Vall previously worked at leading banks such as BNY Mellon, BNP Paribas, UBS Investment Bank, and more. Vall holds a Master’s degree in Quantitative Finance from New York University (NYU), a Certificate in Data and AI from the Massachusetts Institute of Technology (MIT), and a Bachelor’s degree in Mathematical Economics from Syracuse and Pace Universities.
Subscribe to insideAI news for free newsletter.
Join us on Twitter: https://twitter.com/InsideBigData1
Join us on LinkedIn: https://www.linkedin.com/company/insideainews/
Join us on Facebook: https://www.facebook.com/insideAINEWSNOW