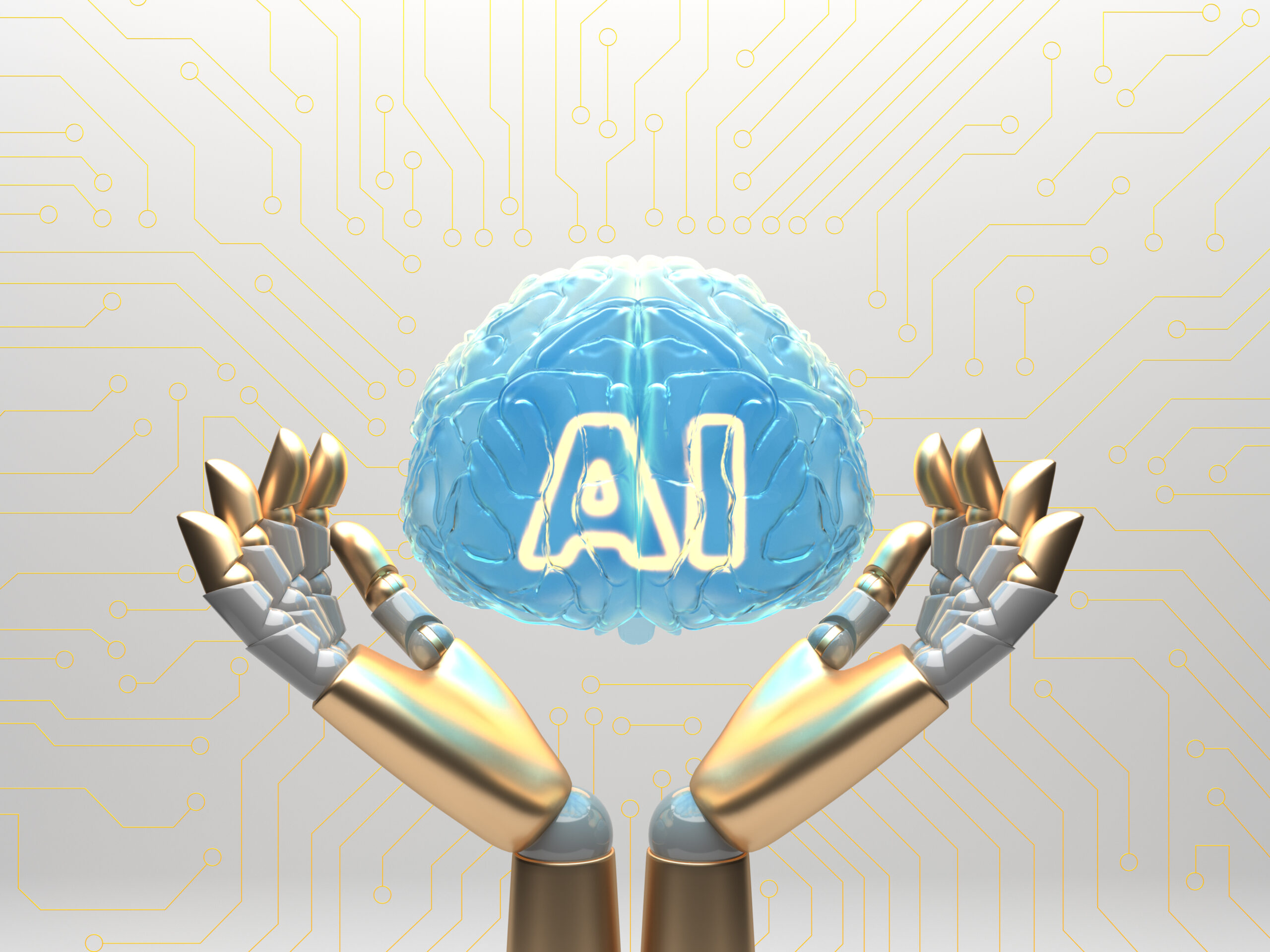
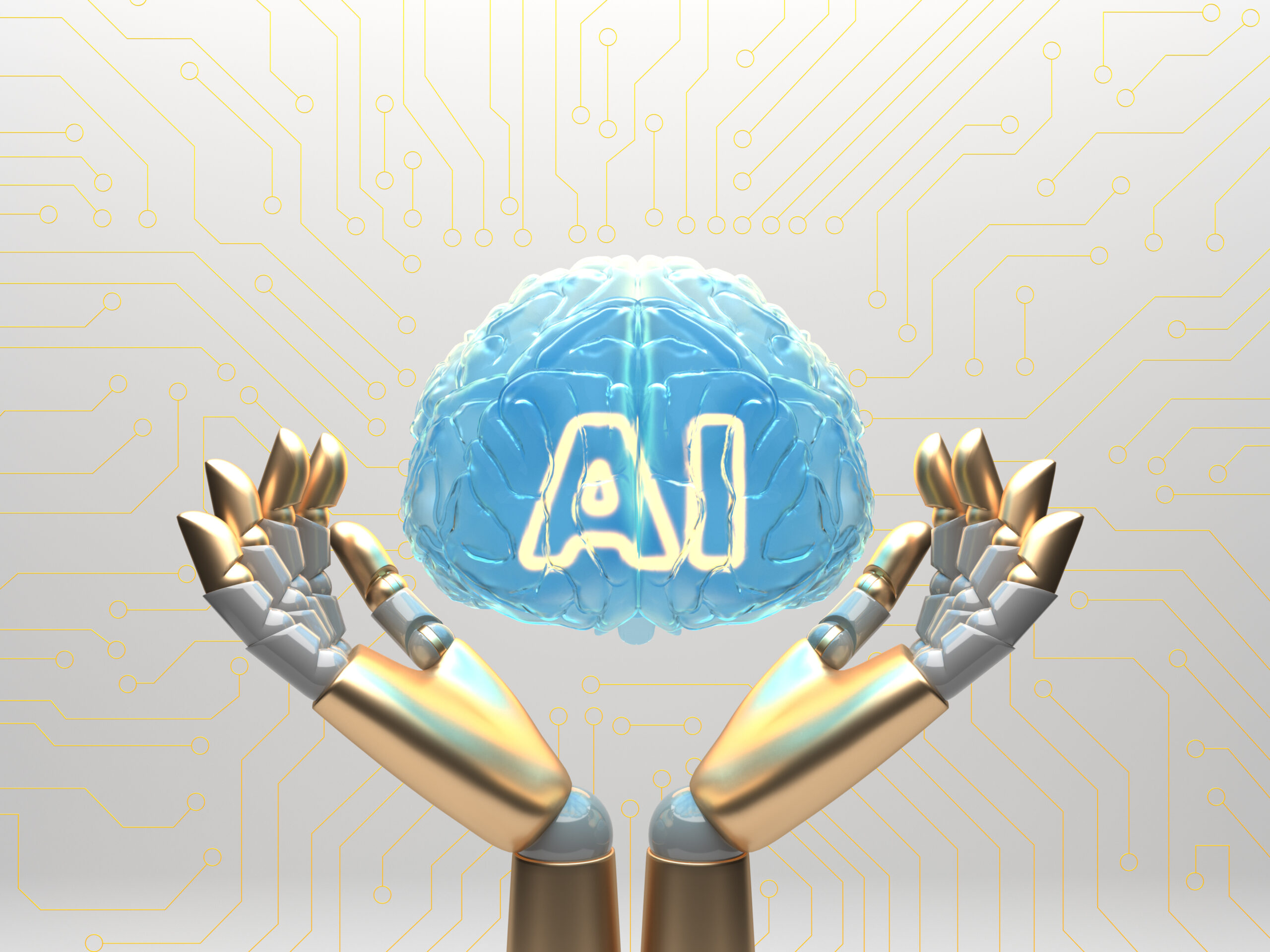
In today’s business market, businesses must constantly seek new ways to take advantage of technological advancements and stay ahead of the curve. Generative AI is an important field that has developed rapidly in recent years.
Gartner predicts that by 2026, more than 80% of organizations will use generative AI APIs, models, or applications, up from less than 5% in 2023. Generative AI has caused a paradigm shift in business analytics. data and associated applications. With just a few quick words, you can receive responses in text, image, audio, or any other format of your choice.
Rather than using traditional AI models to make predictions, this is done by understanding and imitating the structure of the underlying data. Generative artificial intelligence (AI) has grown in just one year thanks to deep learning techniques and applications across many industries.
We will explore the principles and models of generative artificial intelligence (AI) and its uses in Data analysis in more detail through this blog.
Role of Generative AI in Data Analysis
Generative AI has disrupted the data analytics industry, as has other businesses, including software development engineering in testing (SDET). It is crucial for data analysis and visualization, with several facets.
Generative AI has created new avenues for gaining insights from massive and complex data sets, ranging from data processing and cleaning to data visualization.
In the context of data analysis, let’s look at some of the main functions studied by generative AI:
1. Improved preprocessing and data augmentation
Data preparation involves transforming unprocessed data into a format for further analysis. It is a complex, multi-step process involving data standardization, reduction, cleansing, and transformation.
Relying on disparate sources for data collection can lead to disparities in accuracy and caliber. GenAI can transform data and filter defects with enhanced data preparation capabilities.
2. Automate analytics-related tasks
Many business intelligence and data analysis tasks involve repetitive work. Automated programs can complete it, but coding takes time. Generative AI can automate the process.
Chatbots, for example, can write custom automation scripts for data extraction. When collecting data, it can automatically filter relevant information based on specified parameters.
3. Generate data to train models
Generative AI can produce synthetic data that closely mimics the original dataset. It is used in circumstances where data is limited or privacy is protected. Creating synthetic data will make it easier to train machine learning models without revealing sensitive information.
It protects data privacy and allows organizations to use massive datasets for training, resulting in robust models.
Generative AI Features in Data Analytics
Here are some key features of generative AI in data analysis
1. Predictive Analytics
Organizations can use generative AI to analyze large data sets, spot patterns and trends, and produce accurate forecasts. For example, businesses can predict stock prices or customer churn rates to gain insightful insights and identify emerging trends.
2. NLP
The NLP industry has seen a significant upheaval due to generative AI. The ability of generative models to understand and generate text that resembles that of a person opens up a wide range of applications. Translation, content creation and feedback chatbots are some examples.
3. Fraud detection
Compared to real-world data, generative AI can generate data that represents typical behavior, helping to identify fraud and anomalies. It can help businesses reduce risk and guard against fraud across a variety of industries, including retail, healthcare, and finance.
Limitation of Generative AI in Data Analysis
Generative AI has demonstrated remarkable current and potential capabilities. Our way of operating could be different depending on its adoption. However, this comes with obstacles and difficulties.
1. Interpretability
Understanding how huge datasets are trained to generate data using generative AI models powered by neural networks can be difficult.
To explain results and foster user confidence, organizations must ensure that elements such as interpretability and explainability are being developed.
2. Model bias
Biases in the training set can affect generative AI models like conventional machine learning models. Outcomes data with biased input have inconsistencies and accuracy issues.
Organizations should use metrics to achieve fair results, identify bias, and carefully select training datasets to avoid this problem.
3. Ethics
Organizations must ensure that data generation meets ethical standards and legal requirements. AI-generated photos and videos are a big concern these days. There is a need to implement new frameworks and rules to reduce ethical risks.
Best Practices for Generative AI in Data Analytics
1. Quality data
Businesses must ensure that high-quality, diverse data is used to train generative AI models. Data from trusted sources, whether first-party or third-party, may be used. To eliminate inaccurate data and improve data analysis, organizations must also clean and prepare their data.
2. Privacy
When using AI generation, protecting private and sensitive data is essential. Throughout the data analysis process, including data collection, storage and sharing, organizations should identify possible threats to user privacy and take appropriate steps to mitigate them.
3. Data security
Another essential element of best practice when thinking about an ethical strategy for using Gen AI is data security. Gen AI systems must be protected from security risks and keep an eye on illegal access. Other measures to reduce dangers include data encryption and frequent protocol changes.
Real-world examples of generative AI
1. Medical imaging
Data privacy concerns have limited the amount of medical imaging data that healthcare organizations can use to train machine learning algorithms. Real-world data can be replicated in synthetic form using generative AI methods. To improve clinical decision-making and patient outcomes, this facilitates the training of reliable diagnostic models.
2. Recommended products
Retailers can provide specific recommendations to users by analyzing customer data. Generative AI models should be trained using a user’s browsing history and past purchases to provide suggestions specific to their needs. As a result, conversion rates increase and customer satisfaction also increases.
3. Geospatial Analysis
Geospatial analysis can understand the size, construction and condition of properties by extracting structured data from high-resolution images using the power of AI generation. Insurance companies can use this to better manage claims, reduce costs and assess property risks.
Final Thoughts
Generative AI has, like any other industry, brought about a paradigm shift in the field of data analysis. Learn artificial intelligence technologies to stay ahead and improve results has led organizations to experience exponential development in recent years.
The simplicity of user interfaces, which allows you to quickly and easily create high-quality text and images using natural language, is an important factor in the huge buzz around GenAI. Its data generation capability sets it apart from conventional models focused on predictions and classifications.
There are many models of generative AI, including popular techniques such as generative adversarial networks (GAN), variational autoencoders (VAE), and Transformer architecture. ChatGPT, Google BERT, and other technologies leverage the transformer architecture to create extended language models (LLMs) that generate content.
In data analytics, generative AI has applications in predictive analytics, fraud detection, data preparation, and visualization. However, adoption is not without problems. Concerns are raised about ethical issues, bias, data privacy and security, and explainability.
With generative AI, the future of data analysis is very bright. Advances in AI architecture, multimodal techniques, and ethical practices have the potential to expand the scope of generative AI.