In 2023, many technology services firms supported their clients’ interest in generative artificial intelligence (AI) by running proofs of concept to reduce operational costs, complete tasks faster, or improve quality. These pilot programs focused on a variety of topics, including AI assistants for internal search on knowledge portals, high-frequency marketing content generation, sales collateral development, and knowledge interfaces that support customer-facing agents and improve productivity and conversion. Some technology services firms ran dozens or hundreds of these pilots for their clients, some with costs as high as $1 million.
In 2024, these customers are moving beyond the exploration phase and investing to develop successful pilot programs. This year, the focus appears to be on reaping the benefits of these pilots and demonstrating the real business value of AI investments. Bain’s latest Global Generative AI Adoption Survey found that generative AI is a top five priority for 85% of respondents. The percentage of companies planning to spend more than $5 million on generative AI is expected to grow from less than 20% in 2023 to 33% in 2024. Another third of companies said they would spend between $1 million and $5 million on generative AI experiments, up from 15% in 2023.
Better functioning: signal expertise
Across industries, most companies expect their technology services partners to play a key role in these efforts, especially if those providers have already developed expertise in using generative AI internally (to improve their own operations) or in how they deliver services – faster, more efficiently, and with higher quality.
Some of the ways technology services companies are using generative AI include:
- Some IT services companies are using AI to personalize their sales materials and RFP responses by gathering critical technology capabilities and success stories from existing repositories. This can speed up the process and highlight the strengths and successes that are most relevant to specific customers.
- Several players in the technology services industry have developed internal chatbots to support queries from frontline HR and IT departments via a conversational bot. These tools are designed to help employees of client companies access information from data across the entire enterprise.
- Another company is using AI to improve its knowledge management and training by ingesting existing training materials and internal support chats to create a kind of digital twin of internal expert trainers.
Delivering Better: Improving Service
Here are some examples of technology services companies using generative AI to improve their services:
- code generation, documentation and testing, with productivity gains of up to 30%;
- Business process outsourcing, in which service providers working with their clients reimagine processes, combining generative AI with automation to improve productivity, customer satisfaction and solution accuracy; and
- invoice processing, in which a large global outsourcing company demonstrated how to cut processing time in half through redesigned processes, generative AI and automation.
Generative AI’s contributions are expected to unfold in waves, each building on previous achievements (see Figure 1). Consider contact centers: Over the next two to three years, half of non-voice interactions, 25 to 35 percent of simple voice interactions, and about 10 percent of complex voice interactions could be replaced by generative AI.
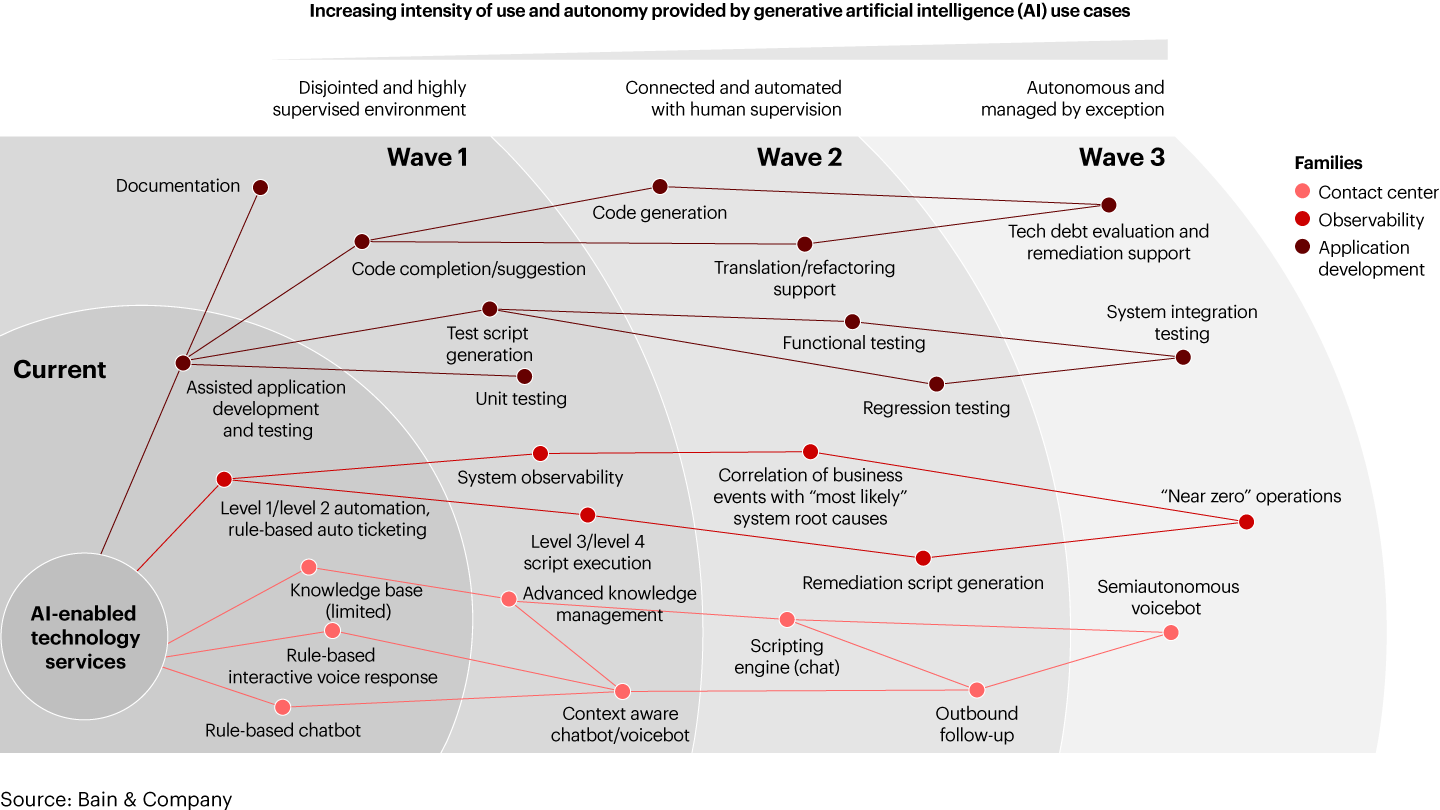
Contact centers have already developed rules-based response engines through chatbots. However, traditional chatbots have limited capabilities to answer specific customer questions or provide customer-specific recommendations, often only offering a link to more information or giving them the option to call them back.
Generative AI’s ability to ingest and synthesize large volumes of unstructured data creates a more accurate knowledge management function. This should enable chatbots and chat assistants to provide faster, more contextual responses to customer queries, leading to happier customers and more productive agents in the first wave of generative AI deployments. In the second wave, generative AI could help develop automated scripts for outbound calls, and in the third wave, we could see semi-autonomous voice bots, both improvements on the first wave rather than basic creations.
Changing the game: moving beyond standalone use cases
Technology services companies looking to design, build, and deploy generative AI solutions tailored to customer needs can differentiate their approach by thinking in terms of collections of use cases, what we call use case families. A family is a set of use cases focused on a group of contiguous processes that need to work in tandem or in sequence and require similar foundational technologies.
Use case families can be horizontal, with nuanced variations across industries, or at the intersection of multiple industries, processes, and geographies. Below are some examples.
- Customer relationship management:Synthesising customer meetings and generating customer outreach materials, including recommendations and social media listening, are examples of a typical horizontal family.
- Outbound Marketing Automation:Micro-segmentation, persona development, best offer prediction (based on usage and purchase knowledge base), awareness content generation, and automated awareness triggers are other horizontal examples of use case families. However, layering nuances related to B2B (persona-driven) or B2C (segment-driven) marketing adds a vertical flavor to this type of family.
- Specific sector families: In financial services, for example, families focused on loan and mortgage origination might include automated pre-approval, application completion, underwriting, processing, disbursement and closing.
Technology services companies can differentiate themselves by selecting and delivering families of use cases that combine their domain expertise, process knowledge, and technical prowess to transform customer engagement modules.
Families are a better approach because standalone use cases cannot necessarily address all the sources of value that can be leveraged with the same technology investment and enablement. Additionally, standalone use cases focus on improving internal productivity, while families of synchronized use cases deliver sustainable customer value, which can lead to repeat purchases, increased sales of the product or service, and new referrals.
Bain research finds that customers are turning to technology services companies to address a few priority families, some promising efficient delivery and others more likely to be game changers (see Figure 2).
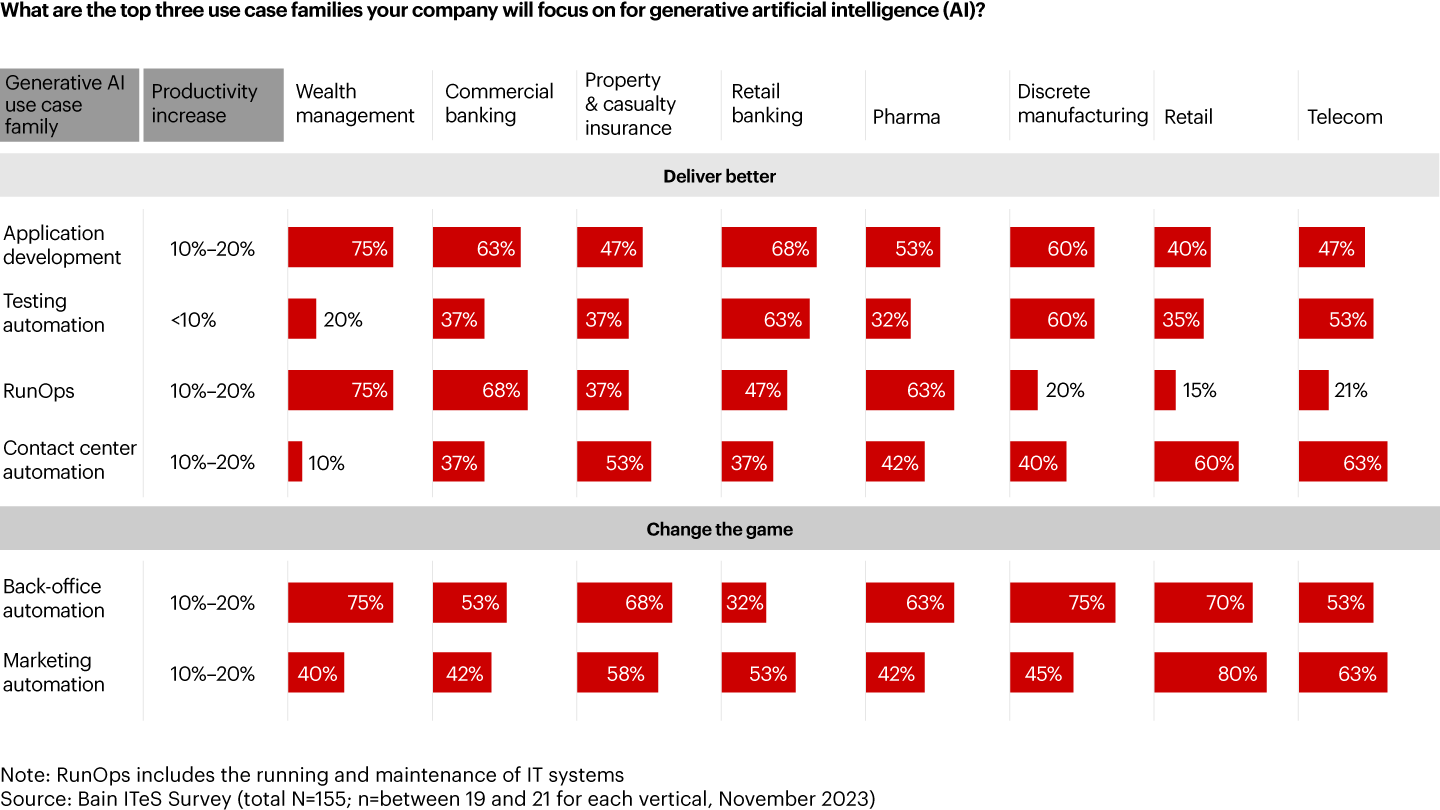
Scaling Generative AI
In our work, we see that clients are eager to partner with technology services players who have already begun to develop their own AI skills and capabilities. Demonstrating expertise, hiring and developing talent to build AI capabilities, and developing and deploying leading-edge solutions are great signals to the market about capabilities and comfort with the technology.
Five additional factors will also differentiate technology services players in the race to become preferred partners in the deployment of generative AI solutions:
- a deep understanding of the customer domain and processes, and a proven track record of innovating and reengineering them through new technological advancements – this has been true for every major technology paradigm shift, such as the advent of robotic process automation and the adoption of the public cloud;
- an ability to articulate, prioritize and sequence use cases to seamlessly realize short- and long-term value;
- Knowledge of technology options (including models, vector databases, and development frameworks) and deployment types (infrastructure and architecture choices) to help customers make the right cost-benefit tradeoffs. Customers often have existing cloud providers with pre-integrated solutions (e.g., Azure AI Platform, including Azure OpenAI Service, and Amazon Bedrock + Anthropic Claude), and therefore want to work with partners who understand how to use these solutions, as well as their advantages and disadvantages;
- model-agnostic accelerators and building blocks, ready to be configured and deployed at scale and speed; and
- a results-based compensation model, which links program success to returns on investment achieved.
Using generative AI to operate and deliver better is now a major challenge for technology service providers. Significant competitive advantage can only come from delivering AI solutions that are game-changing for customers. But this requires not only new skills, but also thinking and focus, because no single provider can be everything to everyone. As technology service providers build the capabilities to operate and deliver better with generative AI, they must also choose where to build the best capabilities to deliver their customers’ full potential.