When storage is discussed in the context of AI or big data analytics, it is assumed to be a high-performance system. In practice, this may not be the case, and the user eventually discovers scalable storage as the amount of data increases. The advantages of having a high-performance storage system then become obvious.
Generative AI and LLMs are now consuming the entire IT world. A large part of the the projector is focused about clustering large amounts of GPUs to train models. Power and cooling issues aside, the storage aspects needed for training data don’t make headlines. Consider the following data point:
The most frequently cited technology inhibitors to AI/ML deployments are data storage and management (35%), significantly more than IT (26%), according to a recent S&P Global Market Intelligence report.
Additionally, it is computationally feasible (although difficult) to perform AI and ML processing without a GPU; However, this is almost impossible without appropriate high-performance storage.
To resolve this problem, a group of experts was brought together to Wall Street HPC+AI Meeting 2024. The panel listed below is composed of two suppliers, an industry consultant and a leading industry analyst.
- Alessandro Petroni, CEO, BusinessMesh Inc.
- Jonathan Symonds, Marketing Director, MinIO
- Molly Presley, senior vice president of global marketing, Hammer space
- Addison Snell, co-founder and CEO, Intersect360 Search
- Douglas Eadline, HPCwire Editor, Moderator
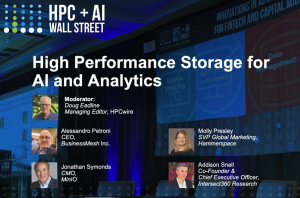
If you have already registered for the show, the entire panel is available on the view website. If you are not registered, a free preview is available. Note that registration is free.
The panel began by asking analyst Addison Snell of Intersect360 Research how you measure the high-performance storage market.
His response was: “This is a complicated and important question. And when we look at the HPC and AI market, the biggest and most important segmentation is not between HPC and AI. This is the most important thing I’m going to say. It’s between hyperscaler and everything else.” Addison’s ongoing discussion presented some important data on the market segment, including Intersect360’s finding that approximately 10% of the overall market is HPC only and no AI, and approximately 10% is AI and no HPC, and the middle 80% is a mix. of people who do both.
The conversation moved to storage in Financial technology And Finservwhich typically have different requirements than traditional HPC storage systems. Industry consultant Alessandro Petroni, BusinessMesh Inc., responded with several issues specific to both areas.
Alessandro explained that there are many legal checks to be made before moving a workload to the cloud. Banks are in the cloud, as is large storage and high performance. Still, it is problematic from a legal perspective and carries a risk for small organizations to use these resources. So it becomes a dilemma. Should I bring my performance to the data center or the cloud? The cloud is always a trade-off between opportunities, risks and rewards.
On the vendor side, storage is often seen as “necessary but not that important until it comes to technology.” The growth of generative AI and the importance of data, even beyond the Big Data wave, has changed this way of thinking. Jonathan Symonds, CMO of MinIO, and Molly Presley of Hammerspace were asked about some critical issues organizations need to consider when implementing “AI-scale” systems.
Jonathan’s response was one of the most memorable comments of the event: “What got you here today is not going to be what you need to get you to where you want to go in the world of ‘AI. And the reason is that the scale this time is fundamentally different. And so the terabyte is the new petabyte.
Other comments have indicated that choosing the right storage technology is critical, because you won’t be able to “fix” or “expand” your system if you get it wrong. Johnathon continued to provide vital storage insights at AI-Scale.
Molly from Hammerspace explained that the vast majority of the budget goes into purchasing the compute, and that’s where the money is made, running the GPUs and getting results. Hammerspace, as a storage company, sees its role as freeing up as much budget as possible for customers. And their approach is not just direct cost, but also power and storage efficiency to ensure those GPUs stay busy.
As an example, Molly cited a Meta case study in which they wanted to ensure they could withstand two outages during the daily operation of their AI cluster, by some estimates containing around 100,000 GPUs; a failure results in downtime and losses that could amount to millions of dollars. The storage simply has to work and work well in this scenario.
Another topic of interest was the adage “treat data where it is” and how this can pose a challenge in many AI computing scenarios. Legacy data may reside on-premises (in existing systems), while other data may exist in the cloud. Due to scalability, GPU compute resources will most likely be in the cloud, but there may also be cases where fine-tuning on-premises makes the most sense. Alessandro provided some thoughts on this question.
He mentioned that this is a big concern, especially when a company starts in R&D mode, eventually piloting and fielding. In R&D mode, the cost of innovation is clear, and the cloud is an accelerator from an economic point of view because you can adapt to changing needs, including GPUs and storage. However, when you begin to operationalize, you will be able to repatriate the workload due to the sensitive nature of the processing. So the decision is: I’m going to buy equipment or rent it one way or another.
Additionally, he mentioned an important item regarding storage capacity versus system performance in the financial community. For the past decade, financial services have been required to retain all data used to make decisions because compliance regulations require it. For example, financial companies must explain how they arrived at a buy or sell recommendation to paying customers.
The conversation continued with a discussion of the well-known rule of thumb in data science: Data acquisition and preparation (or data munging) requires eighty percent of the effort, while the remaining twenty percent involves the actual processing of the data.“A similar claim can be made for generative AI. This process, which takes place before training begins, requires fast, reliable and flexible access to different types of data, potentially in different global locations, on-premises and in the cloud.
Members discussed this and other interesting topics as the panel continued. You can find the full video of the panel as well all other exhibition panels and keynote speakers on the Wall Street HPC+AI site. Free registration is required.