Accelerated adoption of artificial intelligence (AI) is fueling a rapid expansion in both the amount of data stored and the number of processes needed to train and run machine learning models. This is driving an unprecedented thirst for additional computing power. To efficiently manage this power, businesses depend on cloud infrastructures capable of handling giant processes at scale. As a result, operating expenses have skyrocketed. For IT leaders, balancing compelling AI-driven innovation in the cloud with cost efficiency is a daunting challenge.
The Impact of AI on Cloud Costs: Managing the Challenge
AI and machine learning are driving up cloud computing costs in a variety of ways. It takes huge volumes of data and a lot of computing resources to train a high-quality AI model. Once this is done, the data used for training the model must be stored. The rapid pace of AI development also means that models require continuous improvement and retraining to keep up with the latest technological innovations.
Additionally, AI and ML workloads often require specialized hardware (e.g. GPU, NPU, and TPU), which is much more expensive than standard computing resources and requires special associated infrastructure for deployment and maintenance. This drives up costs even more.
Clearly, the computing needs and resource utilization of AI workloads are often at odds with the budget constraints placed on businesses. That’s not all. Fluctuating workloads (calculating multiple images in parallel, for example) that may require a lot of computing power at one time and less at another, can make it difficult to know exactly how much will be needed and when.
Businesses must manage all of this while maintaining the performance and efficiency of AI models and efficiently planning resources to minimize expenses.
AI and ML offer solutions to their own cost problems
The good news? While they put real pressure on costs, AI and ML are also a powerful way to address these pressures, both in the short and long term.
Whether driving automation or optimizing the use of cloud resources, AI is integral to delivering efficient and cost-effective solutions. An example? AI-driven optimization algorithms can dynamically adjust resource levels by leveraging usage patterns and performance metrics to provide computing power when it is needed and reduce it when demand is low.
They can even dynamically scale their capacity to meet changing needs, significantly reducing costs associated with over- and under-provisioning.
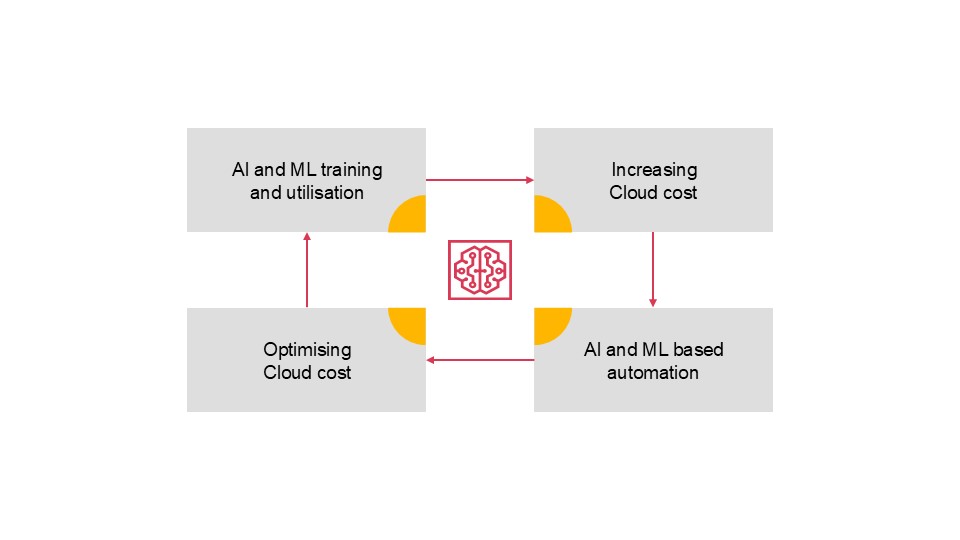
PWC
AI-powered predictive models are essential for forecasting peak usage and scaling resources. By analyzing historical data to identify trends, a model can predict future demand, which can help businesses prepare for peaks in resource usage and avoid costs associated with unused resources during periods of low demand.
Additionally, AI-powered FinOps can provide real-time cloud cost monitoring and management, allowing organizations to better understand and manage their cloud resource consumption. This helps them gain visibility into their usage and identify cost reduction opportunities.
Position the business for lasting success
The advent of FinOps and AI injects a whole new dimension into cost optimization and innovation. Even though AI consumes enormous amounts of computing power and resources, its capabilities, combined with those of FinOps, offer measurable opportunities for savings and resource management – proactively and strategically providing businesses with bandwidth for innovation and growth, while optimizing their operational expenses.
Bottom line: By harnessing the power of AI-driven automation, predictive modeling, and real-time cost tracking, businesses can seize new opportunities to maximize revenue, optimize costs, and improved resource allocation.
Particularly today, as customers and investors place more importance on innovative business solutions that can support innovation and growth through FinOps, this sets the benchmark for improving technology and financial performance , positioning AI-enabled businesses for long-term success in an increasingly competitive landscape. To learn more, visit us here.
To learn more about Nicola Sfondrini, click here.
To learn more about Sebastian Paas, click here.