On a recent trip to Europe, between Vienna and Frankfurt, my plans changed, as they often do on business trips.
I arrived at the airport very early, so I thought maybe I could catch another flight. In the help desk queue, I scanned my boarding pass, took a number, and waited while a few other customers processed their requests. When my turn came, I approached the desk – but before I could even say hello, the assistant said to me in English: “Hello, Mr. Farmer.” You are here quite early; do you want an earlier flight? »
I had barely nodded when she handed me an already printed boarding pass, apologizing for only having a middle seat instead of my preferred window. She then gave me a priority pass through the security line, specifying that I only had a short time before boarding.
The service was perfect: personal, conscious, precise and attentive. This is the kind of service that a top concierge or service agent can provide, but only with a wealth of experience and real personal commitment to their customer. My service agent was good, but what made him great was the data-driven insight and incentives of the customer service system, which I could see her referring to.
For me, as a customer, it didn’t matter that this exchange was data-driven. But predict and resolve my request with minimal fuss and maximum attention to detail? It was delicious: a great example of customer service in the age of personalization.
With the potential for services like this, it’s no surprise that data science and machine learning have become indispensable in modern business workflows, helping to tailor product offerings, predict market trends, and personalize marketing. By using data analytics and algorithms, businesses can gain valuable insights to significantly improve their operations and support.
This data-driven approach represents a fundamental shift. Businesses can now tailor their services to their individual customers in an unprecedented way, which customers increasingly expect.
Data Science Use Cases in Business Workflows
Data science has opened up exciting possibilities for businesses hyper-personalized, tailor-made services. Businesses across many industries use customer and operational data to optimize their operations.
However, businesses must also use data ethically. Through responsible data practices, businesses can unlock the full potential of data in terms of business value and positive social impact. The data-driven business model is ushering in a new era for many industries: the era of personalization.
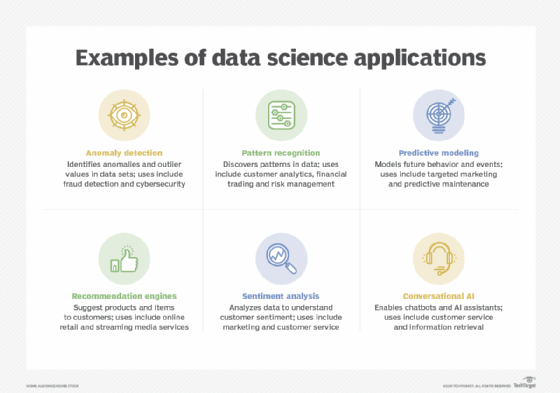
Personalized Marketing and Product Offerings
The era of blanket, one-message-for-all marketing is gradually fading, paving the way for more personalized – and more effective – marketing strategies.
By analyze customer dataretailers can now fine-tune their marketing campaigns, often at the individual consumer level preferences. This transition is not only about improving engagement, but also about optimizing the ROI of marketing campaigns, which has historically been difficult to measure.
However, personalization doesn’t stop at marketing. In the retail industry, data science has transformed the way online sellers present their products to buyers. By reviewing past purchase data, retailers can discover customer preferences and tailor product recommendations accordingly.
For example, online clothing retailer Stitch Fix uses algorithms extensively to learn each customer’s style. This allows Stitch Fix to ship automated deliveries of items they are likely to love and keep directly to customers. Although customers provide some explicit functionality, such as size preferences, the system infers other functionality from data such as purchase histories and Pinterest boards and by use natural language to analyze customer feedback.
Healthcare Improvements and Drug Development
Although the healthcare industry is not as commercial as the retail industry, it still relies on data to improve patient outcomes.
Predictive analytics in healthcare can help providers identify patients at risk of developing certain conditions, such as heart disease, allowing doctors to intervene sooner. The data also allows hospitals to reduce readmission rates by identifying factors associated with hospital readmission. This helps health systems develop targeted care management strategies for specific high-risk patients.
Data science is also useful for pharmaceutical research and development. Machine learning algorithms can now analyze millions of compounds to identify potential drug candidates in just a few days – a process that previously took human researchers months or even years.
Know your customer
In the financial services industry, the Know Your Customer (KYC) framework is not just a suggestion for better service, but an essential regulatory requirement designed to prevent fraud, money laundering and terrorist financing. Data science plays a dual role in KYC, functioning as both a protection measure and a personalization tool.
Financial institutions, by law, must verify the identity of their customers. This usually involves checking official documents such as passports, driving licenses or utility bills. But institutions must also evaluate an account’s purpose, expected transaction behaviors, and sources of funds to ensure they match what is known about the customer.
Data science can be a big help in this regard, especially when operating at the scale of a large consumer bank. Regular monitoring of customer accounts allows financial service providers to detect suspicious activity. Anomalies such as a high-value transaction or unusual transfer pattern can trigger further investigations, making anomaly detection a critical use case for data science in financial services.
With these regulatory elements in place, data science can also extend KYC principles to service offerings. Information about a customer’s behavior and financial needs helps banks and other financial institutions improve their recommendations to that customer for specific products or services. For example, a customer with regular international transactions could benefit from a multi-currency account.
Data Ethical Considerations for Business
Data analytics has become a powerful tool that businesses leverage to drive personalization in design, marketing, and customer service. However, these benefits come with significant ethical challenges.
Often, customers interact with digital platforms without fully understanding the trail of data they leave behind. Companies are responsible for transparency and direct communication on how they collect, store and use customer data. Additionally, companies should provide customers with clear options to opt out of data collection or even request deletion of data.
Yet transparency alone does not end the ethical considerations associated with the use of data in commercial contexts. Bias in algorithms, mainly resulting from biased data entry, can lead to unfair or discriminatory results. For example, a discriminatory algorithm can lead to biased product recommendations or even influence important decisions like loan approval.
Organizations must rigorously audit the data they use to train machine learning algorithms, ensuring representativeness and eliminating unwanted bias. Additionally, continued monitoring of these algorithms after deployment is crucial. It is not enough to define ethical policies: companies must test them in practice.
Donald Farmer is the Director of TreeHive Strategy, where he advises software companies, businesses and investors on data and advanced analytics strategy. He has worked on some of the market’s leading data technologies and at award-winning startups. He previously led design and innovation teams at Microsoft and Qlik.