EEarlier this month, Google launched its long-awaited “Gemini” system, giving users access to its AI image generation technology for the first time. While most early adopters agreed that the system was impressive, creating detailed images for text prompts in seconds, users soon discovered that it was difficult to get the system to generate images of white people, and soon viral tweets displayed striking examples such as Nazis of various races.
Some people have criticized Gemini for being “too woke,” using it as the latest weapon in a growing culture war over the importance of recognizing the effects of historical discrimination. Many said this reflected unease within Google, and some critical the field of “AI ethics” as an embarrassment.
The idea that ethical AI work is responsible is false. In fact, Gemini showed Google did not apply correctly lessons from AI ethics. Where AI ethics focuses on solving predictable use cases – such as historical representations – Gemini seems to have opted for a “one size fits all” approach, resulting in an awkward mix of refreshingly diverse and worthy of interest.
I should know. I’ve been working on AI ethics within technology companies for over 10 years, which makes me one of the most seasoned experts in the world on the subject (it’s a young field!). I also founded and co-led Google’s “Ethical AI” team, before they fired me and my co-lead following our report warning of exactly these kinds of problems for the generation of languages. Many people criticized Google for its decision, saying it reflected systemic discrimination and a prioritization of reckless speed over a well-considered AI strategy. It’s possible, I completely agree.
The Gemini debacle has once again exposed Google’s inexpert strategy in areas where I am uniquely qualified to help and which I can now help the public understand more generally. This article will discuss ways AI companies could do better next time, avoiding giving the far right unnecessary ammunition in the culture wars and ensuring AI benefits as many people as possible. ‘future.
A critical part of implementing ethics in AI is articulating predictable uses, including malicious and abusive uses. This means working on issues such as Once the model we plan to build is deployed, how will people use it? And how can we design it to be as beneficial as possible in these contexts? This approach recognizes the central importance of “context of use” when creating AI systems. This type of foresight and contextual thinking, grounded in the interaction of society and technology, is more difficult for some people than others – this is where people with expertise in human-computer interaction, in social sciences and cognitive sciences are particularly competent (addressing the importance of interdisciplinarity in technological recruitment). These roles tend not to have as much power and influence as engineering roles, and I suspect this was true in Gemini’s case: those most competent at articulating predictable uses were not empowered, this which has led to a system incapable of handling many types of appropriate use, such as the representation of historically white groups.
Things go wrong when organizations treat all use cases as one use case, or don’t model use cases at all. So, without ethical analysis of use cases in different contexts, AI systems might not have “under the hood” models to identify what the user is asking for (and whether it should be generated). For Gemini, this could involve determining whether the user is looking for historical or miscellaneous images, and whether their request is ambiguous or malicious. We recently saw this same failure in building robust models for predictable use, leading to the proliferation of AI-generated Taylor Swift pornography.
To help you, I created the following table years ago. The task is to fill the cells; I filled it today with some examples relevant to Gemini in particular.
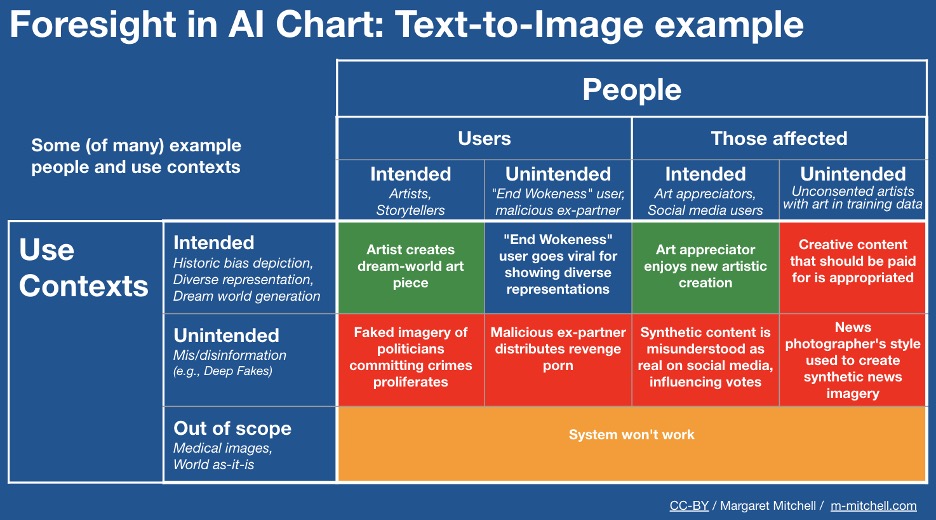
Green cells (top row) are where beneficial AI is most likely possible (not where AI will always be beneficial). The red cells (middle row) are where harmful AI is most likely (but they may also be where unanticipated beneficial innovation can occur). The rest of the cells are more likely to have mixed results – some good, some bad.
Next steps will be to examine likely errors in different contexts, addressing disproportionate errors for subgroups prone to discrimination. The Gemini developers seem to have this part largely figured out. The team appears to have had the foresight to recognize the risk of overrepresentation of white people in neutral or positive situations, which would amplify a problematic white-dominant worldview. And so, there was probably a submodule in Gemini designed to show darker skin tones to users.
The fact that these steps are evident in Gemini, but not in steps involving predictable usage, may be due in part to increased public awareness of bias in AI systems: pro-white bias was a nightmare of easily predictable, echoing public relations the gorilla incident that Google became infamous for, whereas nuanced approaches to managing “context of use” were not. The net effect was a system that “missed the mark” in including appropriate and predictable use cases.
The bottom line is that it is possible to have technology that benefits users and minimizes harm to those who are most likely to be negatively affected. But you need to include people knowledgeable in this area in development and deployment decisions. And these people are often powerless (or worse) when it comes to technology. It doesn’t have to be this way: we can offer different paths to AI that enable the right people to do what they are best qualified to do. Where diverse perspectives are sought out, not closed off. Getting there takes hard work and ruffling feathers. We’ll know we’re on the right track when we start seeing tech leaders as diverse as the images Gemini generates.