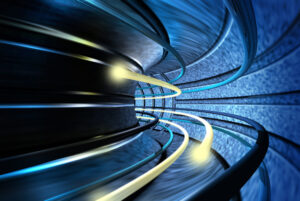
(anterovium/Shutterstock)
The ability to mine, process, and leverage vast volumes of data enables organizations to stand out in today’s data-driven landscape. To stay ahead, businesses must master the complexities of artificial intelligence (AI) data pipelines.
The use of data analytics, BI applications, and data warehouses for structured data is a mature industry, and strategies for extracting value from structured data are well known. However, the emerging explosion of generative AI now promises to extract hidden value from unstructured data as well. Enterprise data often resides in disparate silos, each with its own structure, format, and access protocols. Integrating these diverse data sources is a significant challenge, but a critical first step in building an effective AI data pipeline.
In an ever-changing AI landscape, businesses are constantly striving to harness the full potential of AI-generated insights. The keystone of any successful AI initiative is a robust data pipeline, which ensures that data flows seamlessly from source to insight.
Overcoming Data Silo Barriers to Accelerate AI Pipeline Implementation
The barriers separating unstructured data silos have now become a severe limitation on how quickly IT organizations can implement AI pipelines without costs, governance controls, and complexity spiraling out of control.
Businesses need to be able to leverage their existing data and cannot afford to reorganize existing infrastructure to migrate all of their unstructured data to new platforms to implement AI strategies. AI use cases and technologies are evolving so quickly that data owners need the freedom to pivot at any time to scale up or down or to connect multiple sites to their existing infrastructure, all without disrupting data access for existing users or applications. As diverse as AI use cases are, the common denominator among them is the need to collect data from many different sources and often multiple locations.
The main challenge is that data access, by both humans and AI models, always goes through a file system at some point. But file systems are traditionally integrated into the storage infrastructure. This infrastructure-centric approach means that when data outgrows the storage platform it resides on, or if different performance requirements or cost profiles dictate the use of other types of storage, users and applications must navigate multiple paths to incompatible systems to access their data.
This problem is particularly acute for AI workloads, where a critical first step is to consolidate data from multiple sources to enable a holistic view of all that data. AI workloads need access to the entire data set to classify and/or label files to determine which ones need to be refined to the next step in the process.
At each stage of the AI journey, the data will be refined. This refinement may include cleaning and training large language models (LLMs) or, in some cases, tuning existing LLMs for iterative inference runs to get closer to the desired result. Each stage also requires different compute and storage performance requirements, ranging from slower, less expensive mass storage systems and archives to high-performance, more expensive NVMe storage.
Fragmentation caused by storage-centric locking of file systems at the infrastructure layer is not a new problem unique to AI use cases. For decades, IT professionals have been faced with the choice of overprovisioning their storage infrastructure to solve for the subset of data that requires high performance or paying the “data copy tax” and added complexity of moving file copies between different systems. This long-standing problem is now also evident in AI model training as well as the ETL process.
Separation of the file system from the infrastructure layer
Conventional storage platforms integrate the file system into the infrastructure layer. However, a software-defined solution that is compatible with any on-premises or cloud storage platform from any vendor creates a high-performance, cross-platform, parallel global file system that spans incompatible storage silos across one or more sites.
With the file system decoupled from the underlying infrastructure, automated data orchestration delivers high performance to GPU clusters, AI models, and data engineers. All users and applications in all locations have read/write access to all data, wherever it resides. Not to copies of files, but to the same files via this global, unified metadata control plane.
Empowering IT organizations to automate self-service workflows
Since many industries such as pharmaceuticals, financial services, or biotechnology require archiving of both training data and the resulting models, the ability to automate the placement of this data into low-cost resources is critical. With custom metadata tags that track data provenance, iteration details, and other workflow steps, recalling old model data for reuse or applying a new algorithm is a simple operation that can be automated in the background.
The rapid shift toward integrating AI workloads has created a challenge that exacerbates the silo issues that IT organizations have been facing for years. And the problems have been mounting:
To compete and manage new AI workloads, data access must be seamless across on-premises silos, locations, and clouds, and support very high-performance workloads.
Agility is needed in a dynamic environment where fixed infrastructure can be difficult to scale due to cost or logistics. Therefore, the ability for enterprises to automate data orchestration across siloed resources or quickly access cloud compute and storage resources has become critical.
At the same time, businesses must cost-effectively connect their existing infrastructure to these new distributed resources and ensure that the cost of implementing AI workloads does not crush the expected return.
To meet the many performance requirements of AI pipelines, a new paradigm is needed to effectively bridge the gaps between on-premises and cloud silos. Such a solution requires new technology and a revolutionary approach to take the file system out of the infrastructure layer to enable AI pipelines to use any vendor’s existing infrastructure without compromising results.
About the Author: Molly Presley brings over 15 years of product and marketing experience to her growth marketing leadership experience at the Hammer space Molly has led the marketing organization and strategy for fast-growing, innovative companies such as Pantheon Platform, Qumulo, Quantum Corporation, DataDirect Networks (DDN), and Spectra Logic. She was responsible for the go-to-market strategy for SaaS, hybrid cloud, and data center solutions across a variety of verticals and data-intensive use cases within these companies. At Hammerspace, Molly leads the marketing organization and inspires data creators and consumers to take full advantage of a truly global data environment.
Related articles:
Three Ways to Connect the Dots in a Decentralized Big Data World
Object storage is a “total cop-out,” says Hammerspace CEO. “You’ve all been fooled”
Hammerspace hits the market with its global parallel file system