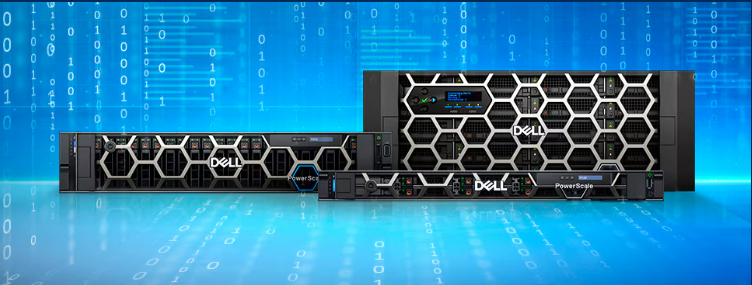
ORDER : Strong data governance is essential for ethical, compliant and effective AI projects. Here’s why the balance between innovation and responsibility is delicate, but crucial.
In a bustling New York office, Emily, a data scientist, races against time. His team is developing an AI algorithm intended to revolutionize personalized customer experiences. The project is ambitious and promising, with the potential to generate unprecedented business growth. However, Emily has one lingering concern: data governance. Despite her enthusiasm, she knows that without strong data governance, the project could face ethical dilemmas, compliance issues and even data breaches. Emily’s story is not unique; This reflects the broader challenges organizations face today when balancing the pursuit of innovation with responsibility for data management.
Artificial intelligence has become the cornerstone of modern innovation, driving advancements in various fields such as healthcare, finance and entertainment. AI’s ability to process and analyze massive amounts of data allows businesses to uncover insights and make decisions that were previously unimaginable. Yet with great power comes great responsibility. The same data that powers AI capabilities also poses significant challenges in terms of governance, privacy, and ethical use.
Data governance is the framework that ensures data is managed correctly throughout its lifecycle. This involves policies, procedures and technologies that maintain data quality, security and compliance. For AI to be truly transformative, organizations must prioritize data governance as much as AI development.
The importance of data governance in AI
As organizations increasingly adopt AI technologies, the need for strong data governance becomes essential. Strong data governance ensures that AI systems are not only efficient and accurate, but also aligned with legal and ethical standards. Here are four crucial ways data governance improves AI projects:
- Ensuring data quality: AI algorithms are only as good as the data they are trained on. Poor quality data leads to inaccurate models, which can lead to flawed business decisions. Data governance ensures that data is accurate, complete and reliable, providing a solid foundation for AI initiatives.
- Compliance and confidentiality: With strict regulations such as GDPR and CCPA, compliance is an essential aspect of data governance. AI projects must comply with these regulations to avoid heavy fines and legal repercussions. Data governance frameworks help organizations manage consent, anonymize data, and implement robust security measures to protect sensitive information.
- Ethical AI: As AI systems become increasingly integrated into decision-making processes, ensuring the ethical use of data is paramount. Data governance provides guidelines to avoid bias, ensure fairness, and maintain transparency of AI algorithms. This not only builds trust with customers but also mitigates risks associated with unethical AI practices.
- Operational efficiency: Effective data governance streamlines data management processes, reducing redundancy and improving efficiency. This allows data scientists and analysts to focus on extracting value from data rather than dealing with data quality issues or compliance obstacles.
Power scale is a storage solution designed to handle huge amounts of unstructured data, making it ideal for AI applications. It’s also a great example of how technology drives and reinforces strong data governance practices with features like:
Scalability and performance
Achieving operational efficiency involves optimizing scalability and performance. PowerScale is designed to scale seamlessly to meet the growing data demands of AI applications while maintaining high-level performance. Based on internal testing that compared PowerScale F910 streaming writing using the OneFS 9.8 distributed file system to PowerScale F900 streaming writing using the OneFS 9.5 distributed file system, the new F910 delivers faster time to AI insights, with a rate of up to 127%. Improved streaming performance (actual results may vary). It accelerates the model checkpointing and training phases of the AI pipeline, keeping GPUs fully utilized with up to 300 PB of storage per cluster. This ensures uninterrupted model training and prevents GPU idling, effectively speeding up the AI pipeline.
Additionally, PowerScale supports GPU Direct and Remote Direct Memory Access (RDMA) technologies, further optimizing data transfer between storage and GPUs. GPU Direct enables direct communication between GPUs and the storage system, bypassing the CPU, reducing latency and improving throughput. RDMA improves this by allowing data to be transferred directly between storage and GPU memory over the network, thereby minimizing CPU involvement and further reducing bottlenecks. Together, these technologies ensure that large data sets are managed efficiently and that data remains accessible and manageable, supporting the development of high-quality AI on our AI-ready data platform.
Data security and compliance
PowerScale integrates advanced security features, including encryption, access controls and audit trails, to protect sensitive data and ensure regulatory compliance. With federal-grade built-in security and real-time API-integrated ransomware detection, it protects the entire AI process from attacks and protects your intellectual property from unauthorized access.
PowerScale also supports isolated environments, providing an additional layer of security by isolating critical systems from insecure networks. This ensures that your most sensitive data remains beyond the reach of external threats, significantly reducing the risk of cyberattacks. The air-insulated configuration is especially crucial for industries with strict compliance requirements, such as finance, healthcare, and government, where data integrity and confidentiality are paramount. By combining seamless protection with comprehensive security measures, PowerScale offers a robust solution that meets the highest standards for data security and regulatory compliance.
Data lifecycle management
PowerScale provides tools to manage data throughout its lifecycle, from creation to archiving, ensuring that data is treated in accordance with governance policies at every stage. This includes not only storage, but also classification, retention and deletion, helping organizations maintain compliance with regulatory requirements. By automating these processes, PowerScale reduces the risk of human error, ensuring that data governance is applied consistently. Additionally, it supports tiering strategies, allowing organizations to move less frequently used data to lower-cost storage while keeping critical data accessible, thereby optimizing cost and performance as workloads grow. of AI are evolving.
Flexibility and integration
PowerScale provides the flexibility to build your infrastructure when, where and how you need it. Its variety of node types and software services allows infrastructure to be scaled and scaled to meet varying workload requirements. Additionally, PowerScale seamlessly integrates with existing data management tools and workflows, including Hadoop Distributed File System (HDFS), NFS, and SMB protocols. For AI-driven workflows, it supports popular data pipeline tools like Apache Spark and TensorFlow. This broad integration capability makes it easy to integrate PowerScale into existing environments, allowing data teams to leverage their current tools while enjoying the scalability and performance benefits that PowerScale offers.
The balance between innovation and responsibility is delicate but crucial. Organizations must foster a culture that values data governance as much as technological progress. This implies:
- Leadership commitment: Leaders must prioritize data governance and allocate resources to develop and maintain strong frameworks. This commitment sets the tone for the entire organization and highlights the importance of responsible data management.
- Cross-functional collaboration: Data governance is not just the responsibility of IT departments. This requires collaboration across all functions, including legal, compliance and business units. This ensures that data governance policies are comprehensive and aligned with the organization’s objectives.
- Continuous improvement: Data governance is an ongoing process that must evolve with changing regulations, technologies and business needs. Regular reviews and updates of governance policies ensure that they remain effective and relevant.
The journey to balance innovation and responsibility is underway. As AI continues to evolve and integrate into various aspects of our lives, the role of data governance becomes increasingly critical. PowerScale illustrates how technology solutions can support this balance, providing the tools needed to manage data efficiently and responsibly.
Ultimately, it’s not just about what AI can achieve, but also how it is implemented. Organizations that prioritize data governance will be better positioned to harness the full potential of AI while maintaining the trust of their stakeholders. Just like the example Emily gave, businesses must recognize that innovation and responsibility go hand in hand, ensuring a future where advances in AI are made with integrity and accountability.
Learn how Dell solutions can help you transform with AI.
Contributed by Dell Technologies.
Kelley Pargmann is a senior product marketing manager for storage and AI at Dell Technologies.