From spell checking to driving cars, artificial intelligence (AI) has become commonplace. AI systems are trained to understand natural language, perceive stimuli, recognize patterns, solve problems, and make decisions. They can learn and adapt from data, improving their performance over time.
At North Carolina State University, interdisciplinary teams are harnessing AI to revolutionize research. Below are several examples of how NC State University researchers, across all colleges and departments, are applying AI to agriculture, chemical engineering, education, and more.
Increasing the value of sweet potatoes with AI
Part of the North Carolina Plant Science Initiative (PSI) and financed by the Breakthrough Research Incentive Program (GRIP), the Sweet-APPS ProjectThis is a transdisciplinary effort using AI to make sweet potato crops more fruitful and profitable. North Carolina is the largest U.S. producer of sweet potatoes—a crop estimated at $324 million in 2019. The research teams used imaging technology to analyze hundreds of thousands of sweet potatoes, calculating their quality characteristics. Combined with data on growing conditions, machine learning algorithms determined which factors impact size and shape with the goal of improving crop yields that meet USDA Grade 1 standards and profitability.
Leveraging AI tools for crops
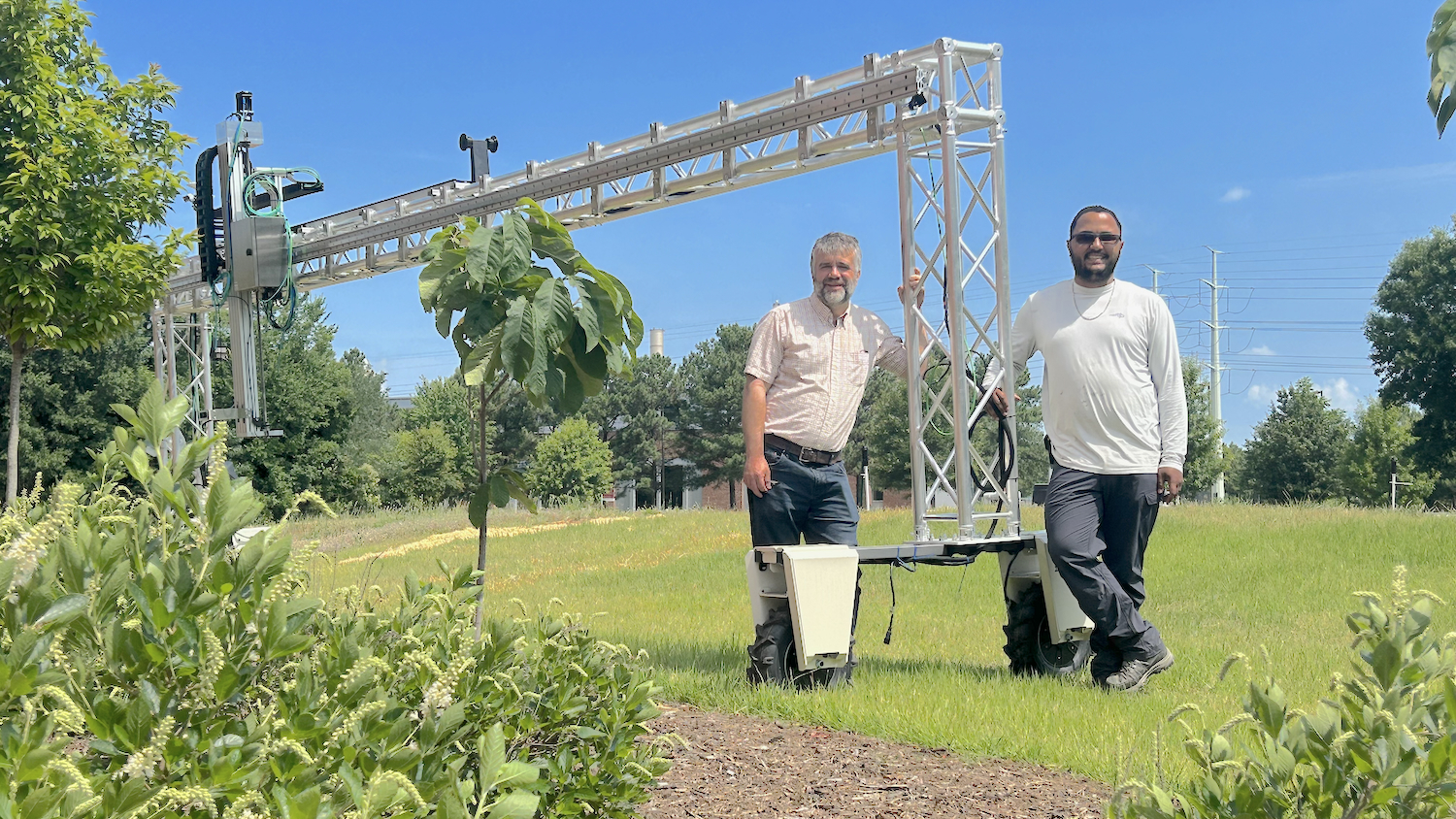
An interdisciplinary research team at the NC Plant Sciences Initiative is using AI to innovate two new tools — BenchBot 3.0 and the NVIDIA Grace Hopper 200 — to help growers and farmers improve crop yield, efficiency and sustainability. BenchBot is a robot that efficiently takes high-resolution photos and stores them in an open-source image repository. Grace Hopper, NC PSI’s newest supercomputer, will process the data collected by BenchBot to help train large-scale AI models for agricultural applications — making them more accurate and predictive.
AI Method Improves Efficiency of Vision Transformers
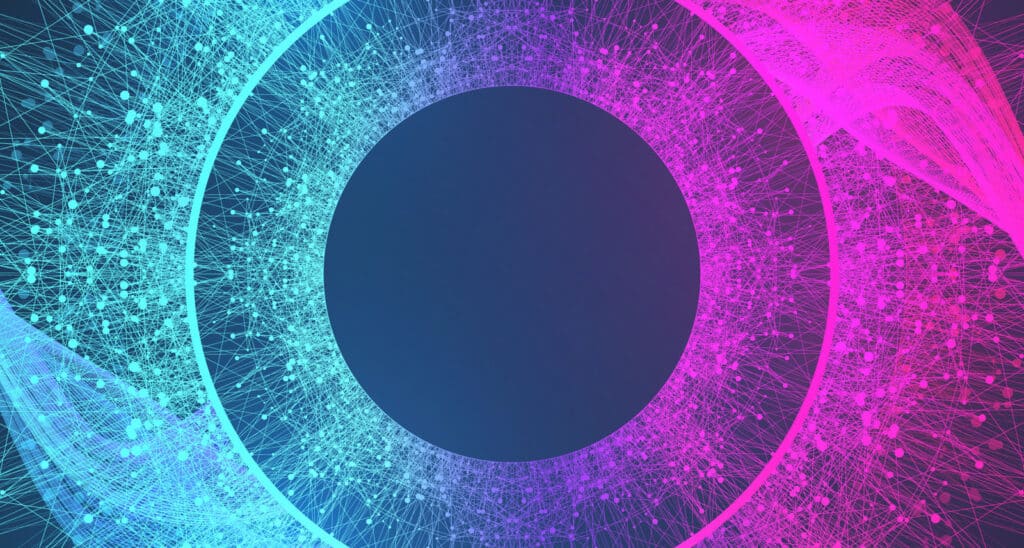
Vision transformers use AI to identify or categorize objects in images, but require significant computing power and memory. Another obstacle is the lack of transparency that users have into vision transformers’ decision-making. “Patch-to-Cluster attention” (PaCa), a new methodology developed by researchers at NC State, addresses both of these challenges and outperforms two advanced vision transformers in their ability to identify, classify, and segment objects in images.
3D space mapping with 2D cameras in self-driving cars
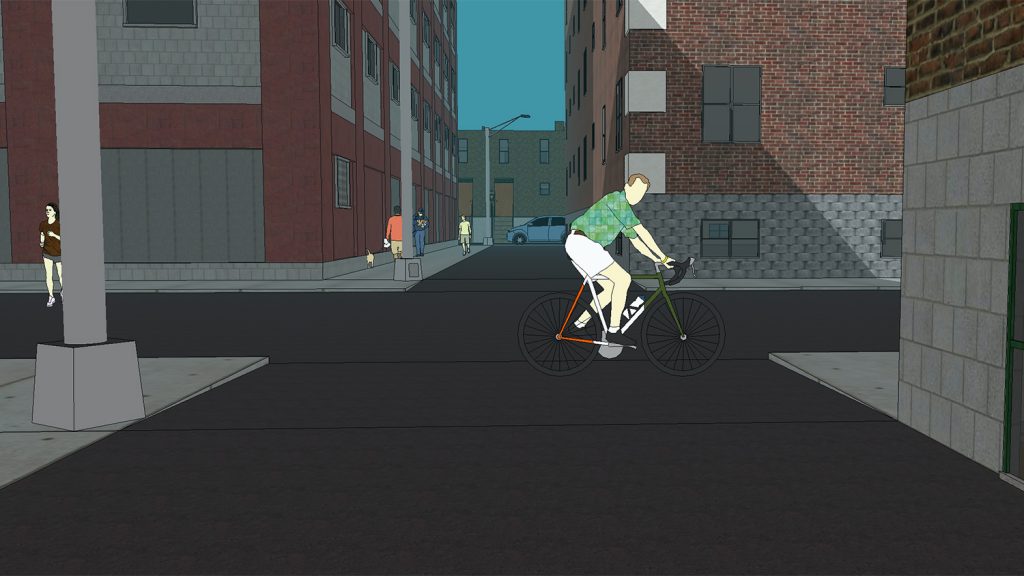
Most self-driving cars rely on multiple 2D cameras that use vision transformers to create a 3D representation of the real-world space around them. Multi-view attentive contextualization (MvACon), launched last year, works by modifying the PaCa approach and allows autonomous vehicles to better map the 3D space around them. A plug-and-play add-on, MvACon works with limited compute resources and is designed to be used with existing vision transformers.
Deciphering age-related disorders
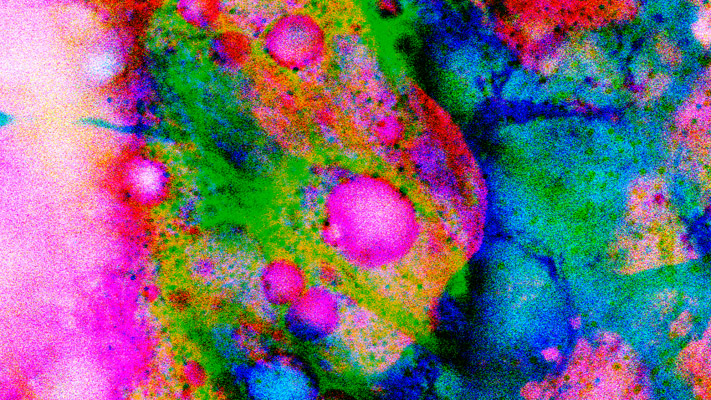
Funded by the Data Science AcademyThe researchers are using worms as a model organism to study aging and age-related diseases. The project, called WormScanAI, aims to decode the complex morphological changes that occur in organs, tissues and cells that are key indicators of underlying age-related disorders. The interdisciplinary team has developed a machine learning algorithm that predicts a worm’s age, but they are still training the algorithm to identify markers of aging or neurodegeneration.
Technique helps AI determine when humans are lying
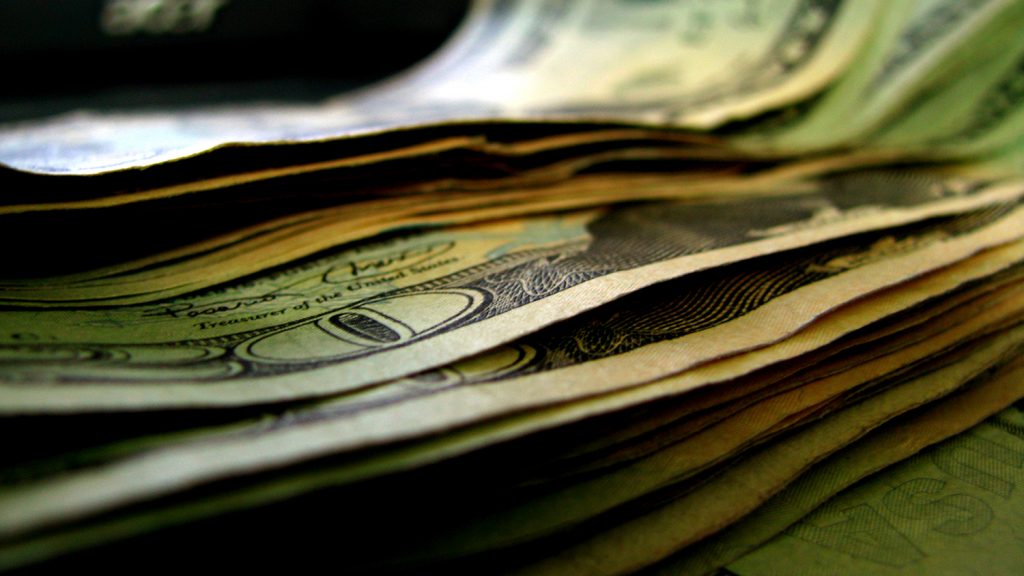
Researchers have developed a new set of training parameters to help AI programs perceive and account for economic incentives to withhold or falsify personal information, such as when applying for a mortgage or trying to lower insurance premiums. The new tool helps train AI to make predictions that account for circumstances in which a human might lie to improve an outcome. The modified AI was able to detect inaccurate information from users in proof-of-concept simulations.
AI-driven lab accelerates catalysis research
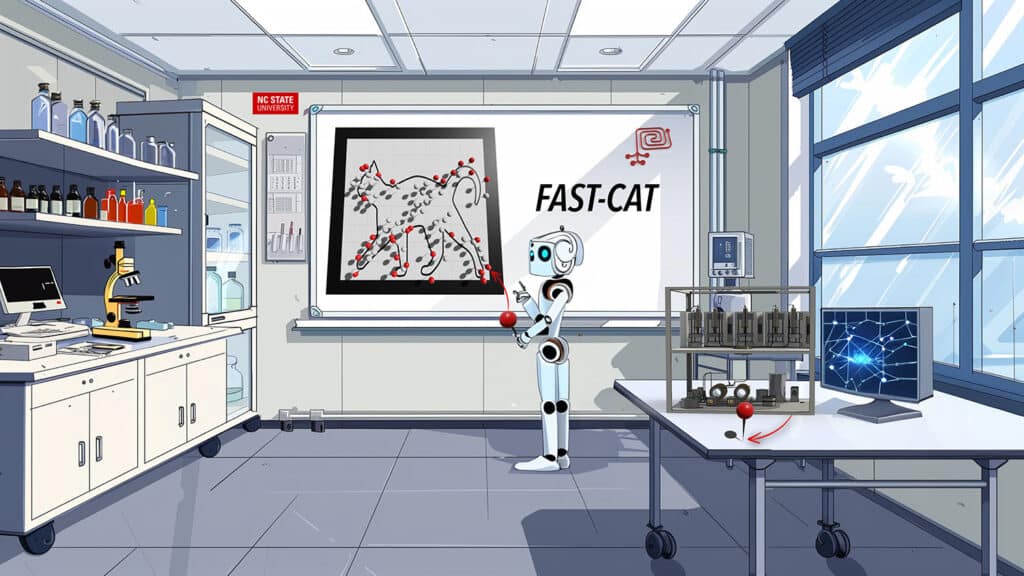
Fast-Cat is an AI tool developed to quickly and efficiently provide in-depth analyses of catalytic reactions in chemical research and manufacturing. The tool streamlines analysis by learning from the automated experiments it runs as an “autonomous” lab. This autonomous technology continuously runs gas-liquid reactions at high temperatures and pressures, then analyzes the output to determine how different variables affect each experimental outcome. The autonomous lab uses these results (both successes and failures) to determine which experiment to run next. It can provide more data than six months of conventional testing in five days.
AI relies on diversity to improve its performance
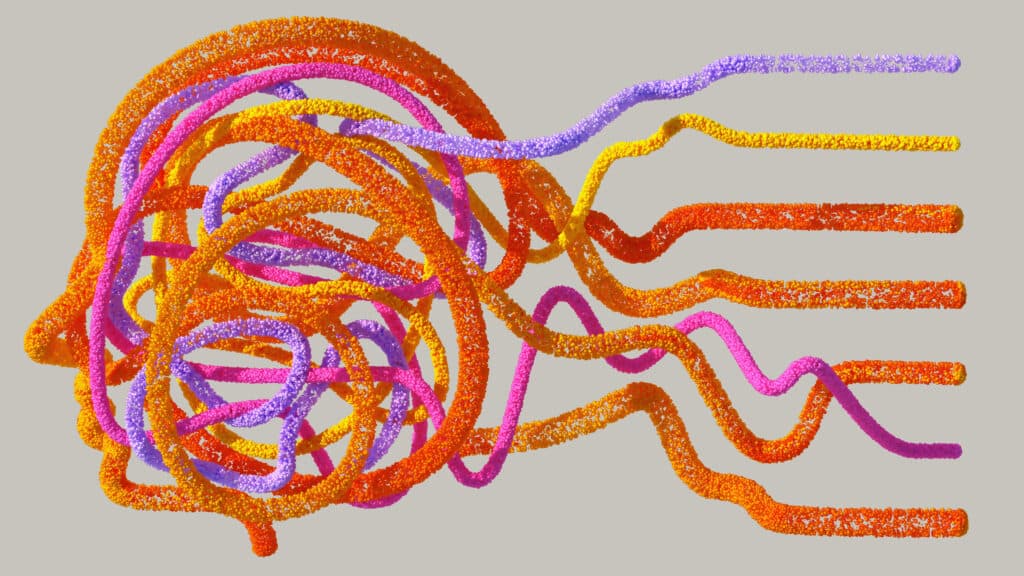
Conventional AI uses neural networks that are typically made up of a large number of identical artificial neurons, unlike a real brain that has different types of neurons. Researchers at North Carolina State University wanted to know what an AI system would choose when given a “control knob” for its own brain, and created one that could look inward and improve its own neural network. If given the choice, it always chose diverse artificial neural pathways over homogeneous pathways to boost its performance.
Advancing the Future of Education with AI
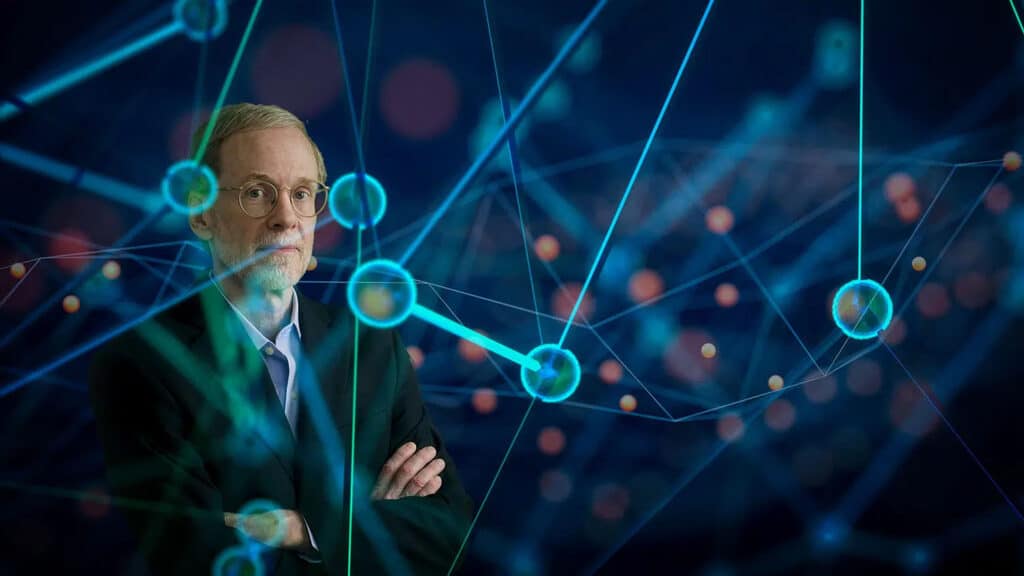
Funded by a five-year, $20 million grant from the National Science Foundation (NSF), a research initiative led by North Carolina State is creating AI tools to advance human learning and education for a broad audience. The NSF AI Institute for Engaged Learning builds on the decades of work that NC State researchers and our collaborators have done to design, develop, and implement AI technologies for education.