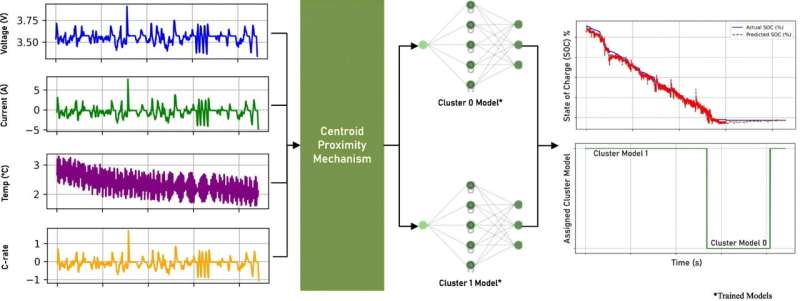
Innovative solution, CBLM. Credit: Energy Storage Journal (2024). DOI: 10.1016/j.est.2024.112866
We are excited to present an advancement in lithium-ion battery performance management, a critical component of the transition to sustainable energy. Our team at the School of Engineering, Technology, and Design at Canterbury Christ Church University, UK, has focused on using machine learning/deep learning to improve the estimation of the state of charge (SOC) of lithium-ion batteries, particularly those that are reused for second-life applications.
The efficient and safe operation of lithium-ion batteries is essential to reduce dependence on fossil fuelspromoting the proliferation of electric vehicles and enabling renewable energy sources to power infrastructure. One of the main challenges in this area is accurate state-of-charge estimation. Poor state-of-charge estimation can lead to overcharging or deep discharge, both of which can significantly degrade battery performance and life.
The Challenge of SOC Estimation
State of charge (SOC) serves as a gauge of how charged a battery is. Just as it is unwise to run out of battery power unexpectedly, it is critical to prevent a battery from discharging or charging beyond safe limits. Accurately estimating the state of charge is essential to ensuring battery longevity and safety, especially in electric vehicles and large-scale energy storage systems.
Our recent study, published in the Energy Storage Journaladdresses this challenge with a novel approach. We developed a cluster-based learning model (CBLM), integrating K-Means clustering with long short-term memory (LSTM) networks. Clustering allows similar data points to be grouped together, facilitating model prediction.
By combining clustering with LSTM, which excels in handling sequence and time series data, the accuracy of SOC estimations is significantly improved. A key feature of this model is the centroid proximity selection mechanism, which dynamically selects the most appropriate cluster model in real time based on battery operational data.
Tests and results
The method was tested using data from a Tesla Model 32,170 lithium-ion battery cell. The results were remarkable, with a root mean square error (RMSE) of 0.65% and a mean absolute error (MAE) of 0.51%. This method outperformed existing techniques by reducing errors by more than 60%, demonstrating its robustness and reliability for real-world applications.
To understand the practical implications, a further examination of the impact of improved state-of-charge estimation on battery health and economic performance was conducted. The CBLM model was compared to the standard LSTM model using second-life electric vehicle battery degradation model in an energy arbitrage application.
The improved SOC estimation method demonstrated significant improvements in maintenance battery The battery is in good operating condition over long periods and under various temperature conditions, including in high depth charge and discharge scenarios. Economically, this method increased profitability over a seven-year period, particularly in high depth discharge scenarios, resulting in substantial cost savings.
Accurate state of charge estimation ensures battery reliability and safety electric vehiclesimproves the efficiency of energy storage systems and facilitates the efficient reuse of end-of-life batteries, thereby extending their life cycle and reducing waste. The adaptability of this approach allows its application to various operational environments, making it a versatile tool in the search for sustainable energy solutions.
This advance marks an important step towards a sustainable energy The future. Collaboration with industrial partners is sought to move this innovation from the laboratory to practical applications. In conclusion, improving state-of-charge estimation contributes to making batteries smarter, more reliable and safer, moving towards a world powered by clean energy.
This story is part of Science X Dialoguewhere researchers can report the results of their published research papers. Visit this page for more information about Science X Dialog and how to participate.
More information:
Mohammed Khalifa Al-Alawi et al., A new and improved method for estimating the state of charge of lithium-ion battery cells using cluster-based LSTM models and centroid proximity selection, Energy Storage Journal (2024). DOI: 10.1016/j.est.2024.112866. is what i.org/10.1016/j.est.2024.112866
Mohammed Al-Alawi is a PhD researcher at Christ Church University, Canterbury, specializing in energy storage and renewable energy engineering. His research focuses on developing sustainable solutions for the reuse of decommissioned electric vehicle batteries, with a focus on improving state-of-charge (SOC) estimation using machine/deep learning techniques. He holds a Master of Science in Renewable Energy Engineering and a Bachelor of Science in Electrical and Electronic Engineering.
Quote: Energizing the Future: AI Innovations for Longer-Lasting Lithium-Ion Batteries (2024, August 21) retrieved August 21, 2024 from https://techxplore.com/news/2024-08-energizing-future-ai-longer-lithium.html
This document is subject to copyright. Apart from any fair dealing for the purpose of private study or research, no part may be reproduced without written permission. The content is provided for informational purposes only.