The world has seen an expansion of AI-based software and robotics in recent years, whether to help with work or for fun, as is the case with Generative AI tools such as ChatGPT and Dall-E. However, AI adoption still lags behind in many industries.
One of the reasons companies are resisting the AI hype is the high cost of AI technologies, as is the case in manufacturing. Other industries are lagging behind in AI adoption, but for different reasons. In agriculture and hospitality, managers are placing more trust in human employees to handle complex and unpredictable situations. This could be a sign of long-term adoption issues.
Industries where AI adoption is lagging
A business leader would justify the upfront costs of expensive AI technology if they hoped to see long-term benefits. If prices are too high or existing processes and employees are sufficient, it is unlikely that a company will want to invest in this new technology. Specific adoption issues vary from industry to industry.
Here are five industries that are experimenting with artificial intelligence but haven’t fully adopted it.
Manufacturing
Robots and AI-based software can come with high costs, and global inflation can make the situation worse. Additionally, AI tools are useful but not ready for critical applications since the datasets are not enough to train them. A 2024 investigation According to ABI Research, 66% of U.S. manufacturers and 62% of German manufacturers have the ability to collect and analyze real-time data. Others lack the ability to produce training data.
Another barriers Are AI systems not integrating well with existing machines and processes used in their factories and are companies being forced to hire new talent who understand AI. Concerns about talent exist in other sectors, including financial services, media and the public sector. Only 20% of respondents in Deloitte’s “State of Generative AI in the Enterprise” study report believe their companies have the talent needed to work with AI systems. This even applies to leaders who are passionate about AI.
Semiconductor factories suffered from global supply chain problems when the COVID-19 pandemic hit. Efforts to repair US supply chains and make chips locally were adopted into lawbut there is still global challenges. Solving these issues is a higher priority than a digital transformation.
Mining
Mining requires a lot of physical activity and takes place in harsh environments. AI-powered machines or collaborative robots (cobots) seen in today’s factories are less reliable over rough terrain and extreme temperatures. In a Goldman Sachs study newsletter published in June 2024, experts believe that what workers do in manufacturing or mining is complex and requires human interaction. AI systems will not catch up or improve any time soon.
Construction
Like other professional services, construction requires human precision that AI cannot provide. Although AI machines are unlikely to be a boon in construction anytime soon, AI software is another matter. AI software can generate designs and schematics more easily, while hardware such as drones monitor sites for security. However, these are just useful tools, and not all construction companies have the budget for this software.
Agriculture
Agriculture, like construction, requires human activity when operating tractors or other machinery. But AI software merged with industrial IoT (IIoT) sensors collect and analyze data on the state of their crops. AI algorithms even predict weather events and the risks that could affect them. Still, farmers see IIoT and AI as useful support tools. Despite the means AI makes farmers’ work easierhuman activity is still needed to do the heavy lifting.
Hospitality
Human empathy – a quality not built into current AI systems – is essential to working in this industry. AI chatbots help workers respond to customer questions and complaints, but are known to current liabilities. They can give customers false hope or promise solutions that don’t exist. These are also called AI hallucinations. Often, a hotel concierge or travel agent is needed to handle guest complaints and complex or nuanced situations.
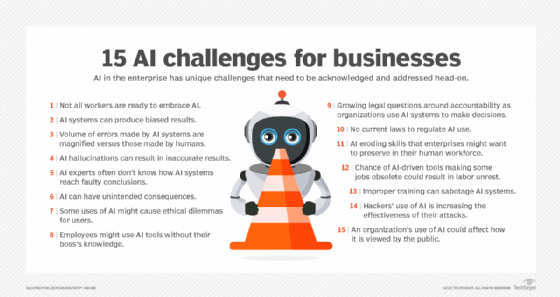
Industries where AI adoption is growing
Despite the reasons for lagging adoption, a few sectors continue to adopt AI. For example, the food industry is compensating for labor shortages with AI robotics. Here, long-term productivity is worth the upfront costs. Automation of an assembly line with robots and cobots powered by machine learning algorithms are now common. These algorithms are good for predictive maintenance. This means monitoring machines to prevent breakdowns before they happen.
Life sciences and telemedicine use cases are there other areas that are not holding back adoption. In life sciences, clinical trials of new drugs can be accelerated using AI as a tool quickly analyzes trial data. This means that new drugs are made available to the public earlier than before. In telemedicine – where healthcare professionals interact with patients virtually – AI can interact with patients to support staff. For example, automating appointment scheduling with AI can alleviate call volumes.
Indications for future trends
Different industries have different reasons for lagging adoption rates. These five examples prove that AI tools are not a silver bullet for every industry. Human precision and empathy are still important, and the imagined final form of AI as seen in science fiction – known as general artificial intelligence – which is supposed to surpass humans in all areas is unlikely in the near future.
But in labor-constrained industries, the long-term benefits of adopting AI for automation could save productivity, making the short-term costs worthwhile. Overcoming resistance will not happen overnight. The hope is that once AI tools become easier to produce and less revolutionary, they will cost less. Even when cost is less of an issue and companies believe their employees are better suited to perform tasks, they might still slowly find new ways to integrate AI tools that would make their employees’ jobs easier.
Cameron Hashemi-Pour is a technology editor for WhatIs. Prior to joining TechTarget, he graduated from the University of Massachusetts Dartmouth and earned a Master of Fine Arts in Professional Writing/Communication. He then worked at Context Labs BV, a software company based in Cambridge, Massachusetts, as a technical editor.